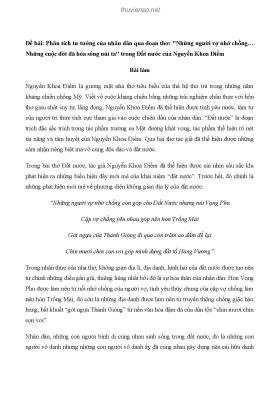
Complex environmental contaminant mixtures and their associations with thyroid hormones using supervised and unsupervised machine learning techniques
Số trang: 9
Loại file: pdf
Dung lượng: 1.30 MB
Lượt xem: 14
Lượt tải: 0
Xem trước 2 trang đầu tiên của tài liệu này:
Thông tin tài liệu:
Bayesian kernel machine regression (BKMR) analysis was used to evaluate the univariate contaminant exposure effect as well as the contaminant mixture effects on levels of thyroid hormones. Significant and positive associations were found between total T3 and PC-2 (high positive nickel and cadmium loadings), total T3 and PC-3 (negative association with negative loading for nickel and positive loading for cadmium) and TSH and PC-1 (high positive loadings for organic contaminants).
Nội dung trích xuất từ tài liệu:
Complex environmental contaminant mixtures and their associations with thyroid hormones using supervised and unsupervised machine learning techniques Environmental Advances 4 (2021) 100054 Contents lists available at ScienceDirect Environmental Advances journal homepage: www.elsevier.com/locate/envadvComplex environmental contaminant mixtures and their associations withthyroid hormones using supervised and unsupervised machine learningtechniquesEric N. Liberda a,∗, Aleksandra M. Zuk b, David S. Di a, Robert J. Moriarity c, Ian D. Martin c,Leonard J.S. Tsuji ca School of Occupational and Public Health and Environmental Applied Science and Management, 350 Victoria St, Ryerson University, Toronto M5B2K3, Ontario,Canadab School of Nursing, Queen’s University, Kingston K7L 3N6, Ontario, Canadac Department of Physical and Environmental Sciences, University of Toronto, Toronto M1C 1A4, Ontario, Canadaa r t i c l e i n f o a b s t r a c tKeywords: Evaluating complex mixtures and their associated health effects poses a challenge in human populations. Herein,Thyroid we assess the association between 17 organic and metal contaminants in blood with thyroid hormones in a remoteMachine learning Indigenous (First Nations) region from Quebec, Canada (n=526). Using principal component analysis (PCA) toContaminants reduce the number of variables, we generated varimax rotated principal component (PC) loadings of contaminantsIndigenous on these uncorrelated synthetic axes. Associations with levels of thyroid hormones (TSH, free T4, and total T3)ExposureBKMR were conducted using multivariable linear regression methods with the participant PC loadings and adjusting forPrincipal component analysis covariates. Additionally, Bayesian kernel machine regression (BKMR) analysis was used to evaluate the univariate contaminant exposure effect as well as the contaminant mixture effects on levels of thyroid hormones. Significant and positive associations were found between total T3 and PC-2 (high positive nickel and cadmium loadings), total T3 and PC-3 (negative association with negative loading for nickel and positive loading for cadmium) and TSH and PC-1 (high positive loadings for organic contaminants). No significant observations were observed for free T4. BKMR provided additional insight into the PCA results and suggested that nickel, and not cadmium, was responsible for driving the observed effects with this effect remaining when evaluating the entire mixture. BKMR analysis did not support the association of TSH with organic contaminants that were found in the PCA regression. Our findings reinforced other studies which showed that metals such as nickel may alter thyroid hormone levels and highlighted how complex environmental mixtures interact with each other. These observations represent an important step to determining how complex mixtures of contaminants can be assessed in human populations, especially those living a subsistence lifestyle who may have high body burdens of contaminants, and to help understand the resultant net effect of exposures on endogenous thyroid hormones utilizing novel machine learning statistical methods.Introduction and carcinogenic effects (Sutcliffe and Harvey, 2015). Metabolic condi- tions, such as hyperthyroidism, have been associated with environmen- There are a variety of xenobiotic compounds th ...
Nội dung trích xuất từ tài liệu:
Complex environmental contaminant mixtures and their associations with thyroid hormones using supervised and unsupervised machine learning techniques Environmental Advances 4 (2021) 100054 Contents lists available at ScienceDirect Environmental Advances journal homepage: www.elsevier.com/locate/envadvComplex environmental contaminant mixtures and their associations withthyroid hormones using supervised and unsupervised machine learningtechniquesEric N. Liberda a,∗, Aleksandra M. Zuk b, David S. Di a, Robert J. Moriarity c, Ian D. Martin c,Leonard J.S. Tsuji ca School of Occupational and Public Health and Environmental Applied Science and Management, 350 Victoria St, Ryerson University, Toronto M5B2K3, Ontario,Canadab School of Nursing, Queen’s University, Kingston K7L 3N6, Ontario, Canadac Department of Physical and Environmental Sciences, University of Toronto, Toronto M1C 1A4, Ontario, Canadaa r t i c l e i n f o a b s t r a c tKeywords: Evaluating complex mixtures and their associated health effects poses a challenge in human populations. Herein,Thyroid we assess the association between 17 organic and metal contaminants in blood with thyroid hormones in a remoteMachine learning Indigenous (First Nations) region from Quebec, Canada (n=526). Using principal component analysis (PCA) toContaminants reduce the number of variables, we generated varimax rotated principal component (PC) loadings of contaminantsIndigenous on these uncorrelated synthetic axes. Associations with levels of thyroid hormones (TSH, free T4, and total T3)ExposureBKMR were conducted using multivariable linear regression methods with the participant PC loadings and adjusting forPrincipal component analysis covariates. Additionally, Bayesian kernel machine regression (BKMR) analysis was used to evaluate the univariate contaminant exposure effect as well as the contaminant mixture effects on levels of thyroid hormones. Significant and positive associations were found between total T3 and PC-2 (high positive nickel and cadmium loadings), total T3 and PC-3 (negative association with negative loading for nickel and positive loading for cadmium) and TSH and PC-1 (high positive loadings for organic contaminants). No significant observations were observed for free T4. BKMR provided additional insight into the PCA results and suggested that nickel, and not cadmium, was responsible for driving the observed effects with this effect remaining when evaluating the entire mixture. BKMR analysis did not support the association of TSH with organic contaminants that were found in the PCA regression. Our findings reinforced other studies which showed that metals such as nickel may alter thyroid hormone levels and highlighted how complex environmental mixtures interact with each other. These observations represent an important step to determining how complex mixtures of contaminants can be assessed in human populations, especially those living a subsistence lifestyle who may have high body burdens of contaminants, and to help understand the resultant net effect of exposures on endogenous thyroid hormones utilizing novel machine learning statistical methods.Introduction and carcinogenic effects (Sutcliffe and Harvey, 2015). Metabolic condi- tions, such as hyperthyroidism, have been associated with environmen- There are a variety of xenobiotic compounds th ...
Tìm kiếm theo từ khóa liên quan:
Environmental advances Environmental contaminant mixtures Thyroid hormones Machine learning techniques Principal component analysis Machine learningGợi ý tài liệu liên quan:
-
9 trang 187 0 0
-
Nuclear energy system's behavior and decision making using machine learning
8 trang 107 0 0 -
19 trang 92 0 0
-
95 trang 62 0 0
-
Ebook Disrupting finance: FinTech and strategy in the 21st century
194 trang 51 0 0 -
Bài giảng Trí tuệ nhân tạo dành cho mọi người - ThS. Nguyễn Ngọc Tú
149 trang 49 0 0 -
Knowledge management system building blocks
12 trang 43 0 0 -
Building credit scoring process in Vietnamese commercial banks using machine learning
14 trang 39 0 0 -
Pseudorandom sequences classification algorithm
8 trang 38 0 0 -
Phát hiện tin giả với python và machine learning
14 trang 35 0 0