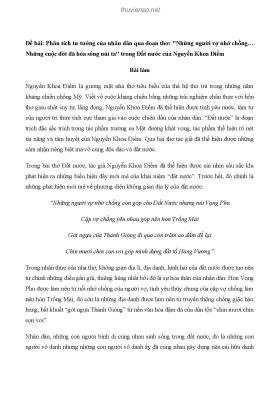
Credit scoring of bank depositor with clustering techniques for supply chain finance
Số trang: 10
Loại file: pdf
Dung lượng: 1,009.20 KB
Lượt xem: 5
Lượt tải: 0
Xem trước 2 trang đầu tiên của tài liệu này:
Thông tin tài liệu:
One of the natural consequences of lending practices by banks and credit institutions has been the creation of deferred and doubtful loans- a phenomenon that has become a major concern for these institutions and has had a negative impact on their revenue and expenditure. From an internal perspective, operating costs, work efficiency, profitability, customer service, branch rank, employee wages and salaries, and other qualitative indicators are significantly affected.
Nội dung trích xuất từ tài liệu:
Credit scoring of bank depositor with clustering techniques for supply chain finance 374 Int. J Sup. Chain. Mg Vol. 8, No. 1, February 2019 Credit Scoring of Bank Depositor with Clustering Techniques for Supply Chain Finance Abdollah Nazari1, Mohammadreza Mehregan 2, Reza Tehrani 3 1 Production and Operations Management, Alborz College, University of Tehran 1nazari_iee@yahoo.com 2,3 Faculty of Management, University of TehranAbstract- One of the natural consequences of lending are as following points. First one is Granting credit to thepractices by banks and credit institutions has been the related parties of the board members and stockholders. Oncreation of deferred and doubtful loans- a phenomenon that the other hand, the data of the improvements in the credithas become a major concern for these institutions and has behavior is updated with a delay. According to the riskhad a negative impact on their revenue and expenditure. knowledge, all related behavior of depositors must beFrom an internal perspective, operating costs, work analyzed in credit scoring and Data of All financialefficiency, profitability, customer service, branch rank,employee wages and salaries, and other qualitative indicators institutions and banks and centers which give creditare significantly affected. From an external perspective, facilities should be analyzed. On the other side, somethese loans lead to slow cash flow, lack of timely and optimal decision making dashboards do not exist and also theallocation of resources to manufacturing networks and amount of risk must be assessed and assigned. Finally, theindustries, low employment rates, and eventually economic amount of collateral should be assigned according to therecession. The purpose of this research is to cluster bank level of risk and of course, there is no informationcustomers and determine the behavioral pattern of each regarding the ability to pay the installment by customerscluster for supply chain finance using K-Means, FCM, and [1].SUB Cluster models in Clementine 18.0, MATLAB 2016, and This study offers a new approach for feature screening inExcel software. 35 models were compared with a variety ofparameters. After removing nonessential variables, the the clustering of massive datasets, in which the number ofmodels were rerun and the outputs for each customer cluster features and the number of observations can be numerous.were provided. The results showed that creditworthiness, Benefitting a fusion penalization based on convexeducation, job, collateral value, collateral type, loan term, clustering criteria, we suggest a highly scalable screeningand age respectively had the greatest impact. Finally, the K- procedure that efficiently discards no informative featuresMeans model was found to be the most appropriate by first computing a clustering score corresponding to theclustering technique. clustering tree constructed for each feature, and then thresholding the resulting values. We present theoreticalKeywords: credit scoring, clustering Techniques, K- support for our methodology by establishing uniform non-Means, FCM, supply chain finance, bank depositor. asymptotic bounds on the clustering scores of the “noise” features. These bounds imply perfect screening of non- informative features with high probability and are derived1. Introduction through careful analysis of the empirical processes corresponding to the clustering trees that are constructedBanks and financial institutions are intermediary for each of the features by the associated clusteringinstitutions which receive money from customers having procedure [2].extra money and allocate it to the depositors looking for Clustering is the learning where the items are grouped onmoney for supply chain finance. The precision of their the basis of some inherent similarity. There are differentdecision has so many degrees of importance for their methods for clustering the objects such as hierarchical,business. Then Assessment of the credit worthiness is so partitional, grid, density based and model based [3].important and they look for different quantitative models During the last two decades, the financial crisis in thein order to reach to the credit scoring of their customers. banking syst ...
Nội dung trích xuất từ tài liệu:
Credit scoring of bank depositor with clustering techniques for supply chain finance 374 Int. J Sup. Chain. Mg Vol. 8, No. 1, February 2019 Credit Scoring of Bank Depositor with Clustering Techniques for Supply Chain Finance Abdollah Nazari1, Mohammadreza Mehregan 2, Reza Tehrani 3 1 Production and Operations Management, Alborz College, University of Tehran 1nazari_iee@yahoo.com 2,3 Faculty of Management, University of TehranAbstract- One of the natural consequences of lending are as following points. First one is Granting credit to thepractices by banks and credit institutions has been the related parties of the board members and stockholders. Oncreation of deferred and doubtful loans- a phenomenon that the other hand, the data of the improvements in the credithas become a major concern for these institutions and has behavior is updated with a delay. According to the riskhad a negative impact on their revenue and expenditure. knowledge, all related behavior of depositors must beFrom an internal perspective, operating costs, work analyzed in credit scoring and Data of All financialefficiency, profitability, customer service, branch rank,employee wages and salaries, and other qualitative indicators institutions and banks and centers which give creditare significantly affected. From an external perspective, facilities should be analyzed. On the other side, somethese loans lead to slow cash flow, lack of timely and optimal decision making dashboards do not exist and also theallocation of resources to manufacturing networks and amount of risk must be assessed and assigned. Finally, theindustries, low employment rates, and eventually economic amount of collateral should be assigned according to therecession. The purpose of this research is to cluster bank level of risk and of course, there is no informationcustomers and determine the behavioral pattern of each regarding the ability to pay the installment by customerscluster for supply chain finance using K-Means, FCM, and [1].SUB Cluster models in Clementine 18.0, MATLAB 2016, and This study offers a new approach for feature screening inExcel software. 35 models were compared with a variety ofparameters. After removing nonessential variables, the the clustering of massive datasets, in which the number ofmodels were rerun and the outputs for each customer cluster features and the number of observations can be numerous.were provided. The results showed that creditworthiness, Benefitting a fusion penalization based on convexeducation, job, collateral value, collateral type, loan term, clustering criteria, we suggest a highly scalable screeningand age respectively had the greatest impact. Finally, the K- procedure that efficiently discards no informative featuresMeans model was found to be the most appropriate by first computing a clustering score corresponding to theclustering technique. clustering tree constructed for each feature, and then thresholding the resulting values. We present theoreticalKeywords: credit scoring, clustering Techniques, K- support for our methodology by establishing uniform non-Means, FCM, supply chain finance, bank depositor. asymptotic bounds on the clustering scores of the “noise” features. These bounds imply perfect screening of non- informative features with high probability and are derived1. Introduction through careful analysis of the empirical processes corresponding to the clustering trees that are constructedBanks and financial institutions are intermediary for each of the features by the associated clusteringinstitutions which receive money from customers having procedure [2].extra money and allocate it to the depositors looking for Clustering is the learning where the items are grouped onmoney for supply chain finance. The precision of their the basis of some inherent similarity. There are differentdecision has so many degrees of importance for their methods for clustering the objects such as hierarchical,business. Then Assessment of the credit worthiness is so partitional, grid, density based and model based [3].important and they look for different quantitative models During the last two decades, the financial crisis in thein order to reach to the credit scoring of their customers. banking syst ...
Tìm kiếm theo từ khóa liên quan:
Credit scoring Clustering techniques Supply chain finance Bank depositor Supply chain finance using K-Means Supply chain managementTài liệu liên quan:
-
14 trang 199 0 0
-
Ebook Small business management: Launching and growing entrepreneurial ventures – Part 2
400 trang 160 0 0 -
Ebook Purchasing and supply chain management: Strategies and realities (1st ed) – Part 1
135 trang 136 0 0 -
Ebook Effective small business management: An entrepreneurial approach (Tenth edition) – Part 2
421 trang 135 0 0 -
Ebook Purchasing and supply chain management: Strategies and realities (1st ed) – Part 2
243 trang 126 0 0 -
Ebook Principles of operations management - Sustainability and supply chain management: Part 1
479 trang 92 2 0 -
Bài giảng Quản lý chuỗi cung ứng (Supply Chain Management): Chương 2 - Đường Võ Hùng
28 trang 75 0 0 -
210 trang 56 0 0
-
Ebook Operations management (7/E): Part 2
495 trang 50 0 0 -
Ebook E-business and e-commerce management: Strategy, implementation and practice - Part 2
514 trang 49 0 0