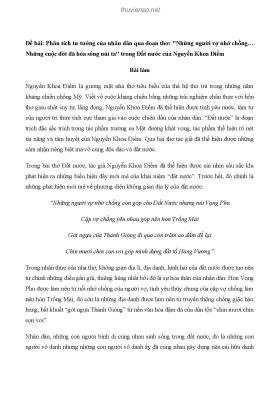
Data Mining Anomaly Detection
Số trang: 25
Loại file: ppt
Dung lượng: 347.00 KB
Lượt xem: 17
Lượt tải: 0
Xem trước 3 trang đầu tiên của tài liệu này:
Thông tin tài liệu:
What are anomalies/outliers?The set of data points that are considerably different than the remainder of the dataVariants of Anomaly/Outlier Detection ProblemsGiven a database D, find all the data points x D with anomaly scores greater than some threshold tGiven a database D, find all the data points x D having the top-n largest anomaly scores f(x)Given a database D, containing mostly normal (but unlabeled) data points, and a test point x, compute the anomaly score of x with respect to DApplications: Credit card fraud detection, telecommunication fraud detection, network intrusion detection, fault detection...
Nội dung trích xuất từ tài liệu:
Data Mining Anomaly Detection Data Mining Anomaly Detection Lecture Notes for Chapter 10 Introduction to Data Mining by Tan, Steinbach, Kumar© Tan,Steinbach, Kumar Introduction to Data Mining 1Anomaly/Outlier DetectionWhat are anomalies/outliers? – The set of data points that are considerably different than the remainder of the dataVariants of Anomaly/Outlier Detection Problems – Given a database D, find all the data points x ∈ D with anomaly scores greater than some threshold t – Given a database D, find all the data points x ∈ D having the top- n largest anomaly scores f(x) – Given a database D, containing mostly normal (but unlabeled) data points, and a test point x, compute the anomaly score of x with respect to DApplications: – Credit card fraud detection, telecommunication fraud detection, network intrusion detection, fault detection © Tan,Steinbach, Kumar Introduction to Data Mining 2Importance of Anomaly DetectionOzone Depletion HistoryIn 1985 three researchers (Farman, Gardinar and Shanklin) were puzzled by data gathered by the British Antarctic Survey showing that ozone levels for Antarctica had dropped 10% below normal levelsWhy did the Nimbus 7 satellite, which had instruments aboard for recording ozone levels, not record similarly low ozone concentrations?The ozone concentrations recorded by the satellite were so low they were being treated as outliers by a computer program and discarded! Sources: http://exploringdata.cqu.edu.au/ozone.html http://www.epa.gov/ozone/science/hole/size.html © Tan,Steinbach, Kumar Introduction to Data Mining 3Anomaly DetectionChallenges – How many outliers are there in the data? – Method is unsupervised • Validation can be quite challenging (just like for clustering) – Finding needle in a haystackWorking assumption: – There are considerably more “normal” observations than “abnormal” observations (outliers/anomalies) in the data © Tan,Steinbach, Kumar Introduction to Data Mining 4Anomaly Detection Schemes General Steps – Build a profile of the “normal” behavior • Profile can be patterns or summary statistics for the overall population – Use the “normal” profile to detect anomalies • Anomalies are observations whose characteristics differ significantly from the normal profile Types of anomaly detection schemes – Graphical & Statistical-based – Distance-based – Model-based © Tan,Steinbach, Kumar Introduction to Data Mining 5Graphical ApproachesBoxplot (1-D), Scatter plot (2-D), Spin plot (3-D)Limitations – Time consuming – Subjective © Tan,Steinbach, Kumar Introduction to Data Mining 6Convex Hull MethodExtreme points are assumed to be outliersUse convex hull method to detect extreme valuesWhat if the outlier occurs in the middle of the data? © Tan,Steinbach, Kumar Introduction to Data Mining 7Statistical ApproachesAssume a parametric model describing the distribution of the data (e.g., normal distribution)Apply a statistical test that depends on – Data distribution – Parameter of distribution (e.g., mean, variance) – Number of expected outliers (confidence limit) © Tan,Steinbach, Kumar Introduction to Data Mining 8Grubbs’ TestDetect outliers in univariate dataAssume data comes from normal distributionDetects one outlier at a time, remove the outlier, and repeat – H0: There is no outlier in data – HA: There is at least one max X − X outlier G= sGrubbs’ test statistic:Reject H0 if: ( N − 1) t (2α / N , N − 2 ) G> N N − 2 + t (2α / N , N − 2 ) © Tan,Steinbach, Kumar Introduction to Data Mining 9Statistical-based – Likelihood ApproachAssume the data set D contains samples from a mixture of two probability distributions: – M (majority distribution) – A (anomalous distribution)General Approach: – Initially, assume all the data points belong to M – Let Lt(D) be the log likelihood of D at time t – For each point xt that belongs to M, move it to A • Let Lt+1 (D) be the new log likelihood. • Compute the differen ...
Nội dung trích xuất từ tài liệu:
Data Mining Anomaly Detection Data Mining Anomaly Detection Lecture Notes for Chapter 10 Introduction to Data Mining by Tan, Steinbach, Kumar© Tan,Steinbach, Kumar Introduction to Data Mining 1Anomaly/Outlier DetectionWhat are anomalies/outliers? – The set of data points that are considerably different than the remainder of the dataVariants of Anomaly/Outlier Detection Problems – Given a database D, find all the data points x ∈ D with anomaly scores greater than some threshold t – Given a database D, find all the data points x ∈ D having the top- n largest anomaly scores f(x) – Given a database D, containing mostly normal (but unlabeled) data points, and a test point x, compute the anomaly score of x with respect to DApplications: – Credit card fraud detection, telecommunication fraud detection, network intrusion detection, fault detection © Tan,Steinbach, Kumar Introduction to Data Mining 2Importance of Anomaly DetectionOzone Depletion HistoryIn 1985 three researchers (Farman, Gardinar and Shanklin) were puzzled by data gathered by the British Antarctic Survey showing that ozone levels for Antarctica had dropped 10% below normal levelsWhy did the Nimbus 7 satellite, which had instruments aboard for recording ozone levels, not record similarly low ozone concentrations?The ozone concentrations recorded by the satellite were so low they were being treated as outliers by a computer program and discarded! Sources: http://exploringdata.cqu.edu.au/ozone.html http://www.epa.gov/ozone/science/hole/size.html © Tan,Steinbach, Kumar Introduction to Data Mining 3Anomaly DetectionChallenges – How many outliers are there in the data? – Method is unsupervised • Validation can be quite challenging (just like for clustering) – Finding needle in a haystackWorking assumption: – There are considerably more “normal” observations than “abnormal” observations (outliers/anomalies) in the data © Tan,Steinbach, Kumar Introduction to Data Mining 4Anomaly Detection Schemes General Steps – Build a profile of the “normal” behavior • Profile can be patterns or summary statistics for the overall population – Use the “normal” profile to detect anomalies • Anomalies are observations whose characteristics differ significantly from the normal profile Types of anomaly detection schemes – Graphical & Statistical-based – Distance-based – Model-based © Tan,Steinbach, Kumar Introduction to Data Mining 5Graphical ApproachesBoxplot (1-D), Scatter plot (2-D), Spin plot (3-D)Limitations – Time consuming – Subjective © Tan,Steinbach, Kumar Introduction to Data Mining 6Convex Hull MethodExtreme points are assumed to be outliersUse convex hull method to detect extreme valuesWhat if the outlier occurs in the middle of the data? © Tan,Steinbach, Kumar Introduction to Data Mining 7Statistical ApproachesAssume a parametric model describing the distribution of the data (e.g., normal distribution)Apply a statistical test that depends on – Data distribution – Parameter of distribution (e.g., mean, variance) – Number of expected outliers (confidence limit) © Tan,Steinbach, Kumar Introduction to Data Mining 8Grubbs’ TestDetect outliers in univariate dataAssume data comes from normal distributionDetects one outlier at a time, remove the outlier, and repeat – H0: There is no outlier in data – HA: There is at least one max X − X outlier G= sGrubbs’ test statistic:Reject H0 if: ( N − 1) t (2α / N , N − 2 ) G> N N − 2 + t (2α / N , N − 2 ) © Tan,Steinbach, Kumar Introduction to Data Mining 9Statistical-based – Likelihood ApproachAssume the data set D contains samples from a mixture of two probability distributions: – M (majority distribution) – A (anomalous distribution)General Approach: – Initially, assume all the data points belong to M – Let Lt(D) be the log likelihood of D at time t – For each point xt that belongs to M, move it to A • Let Lt+1 (D) be the new log likelihood. • Compute the differen ...
Tìm kiếm theo từ khóa liên quan:
Data Mining Alternative Techniques training data Simple models Logistic regression Decision treesGợi ý tài liệu liên quan:
-
17 trang 186 0 0
-
Impact of macro and regional fators on the SMEs' investment in Northwest Vietnam
16 trang 46 0 0 -
Bài giảng Khai phá dữ liệu (Data mining): Chương 8 - ĐH Bách khoa TP.HCM
8 trang 45 0 0 -
60 trang 41 0 0
-
Bài giảng Khai phá dữ liệu (Data mining): Clustering - Trịnh Tấn Đạt
70 trang 41 0 0 -
Lecture Data mining: Lesson 19
27 trang 41 0 0 -
Ebook Symbolic data analysis and the SODAS software
478 trang 41 0 0 -
36 trang 39 0 0
-
25 trang 38 0 0
-
Ebook Introduction to machine learning
209 trang 38 0 0 -
Ebook Data warehousing and data mining
88 trang 38 0 0 -
49 trang 37 0 0
-
Lecture Data mining: Lesson 22
87 trang 37 0 0 -
Bài giảng Khai phá dữ liệu (Data mining): Data preprocessing - Trịnh Tấn Đạt
71 trang 36 0 0 -
48 trang 34 0 0
-
Bài giảng Khai phá dữ liệu (Data mining): Linear regression - Trịnh Tấn Đạt
64 trang 34 0 0 -
Partition fuzzy domain with multi granularity representation data based on hedge algebra approach
13 trang 34 0 0 -
Lecture Data mining: Lesson 21
20 trang 33 0 0 -
169 trang 33 0 0
-
Ebook Data mining - Know it all
477 trang 33 0 0