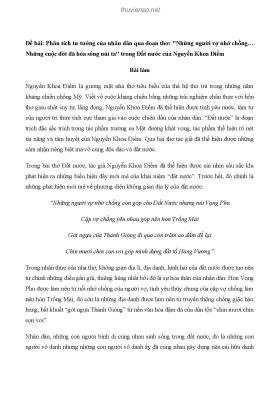
Development of hybrid model and optimization of surface roughness in electric discharge machining using artificial neural networks and genetic algorithm
Số trang: 9
Loại file: pdf
Dung lượng: 490.54 KB
Lượt xem: 12
Lượt tải: 0
Xem trước 2 trang đầu tiên của tài liệu này:
Thông tin tài liệu:
(BQ) The present work is aimed at optimizing the surface roughness of die sinking electric discharge machining (EDM) by considering the simultaneous affect of various input parameters.
Nội dung trích xuất từ tài liệu:
Development of hybrid model and optimization of surface roughness in electric discharge machining using artificial neural networks and genetic algorithmj o u r n a l o f m a t e r i a l s p r o c e s s i n g t e c h n o l o g y 2 0 9 ( 2 0 0 9 ) 1512–1520journal homepage: www.elsevier.com/locate/jmatprotecDevelopment of hybrid model and optimization of surfaceroughness in electric discharge machining using artificialneural networks and genetic algorithmG. Krishna Mohana Rao a,∗ , G. Rangajanardhaa b ,D. Hanumantha Rao c , M. Sreenivasa Rao aabcJNTU College of Engineering, Hyderabad 85, AP, IndiaDepartment of Mechanical Engineering, Hoseo University, South KoreaDeccan College of Engineering and Technology, Hyderabad, AP, Indiaa r t i c l ei n f oa b s t r a c tArticle history:The present work is aimed at optimizing the surface roughness of die sinking electric dis-Received 27 August 2007charge machining (EDM) by considering the simultaneous affect of various input parameters.Received in revised formThe experiments are carried out on Ti6Al4V, HE15, 15CDV6 and M-250. Experiments were28 March 2008conducted by varying the peak current and voltage and the corresponding values of surfaceAccepted 2 April 2008roughness (SR) were measured. Multiperceptron neural network models were developedusing Neuro Solutions package. Genetic algorithm concept is used to optimize the weighting factors of the network. It is observed that the developed model is within the limits of theKeywords:agreeable error when experimental and network model results are compared. It is furtherEDMobserved that the error when the network is optimized by genetic algorithm has come downSurface roughnessto less than 2% from more than 5%. Sensitivity analysis is also done to find the relative influ-Hybrid modelence of factors on the performance measures. It is observed that type of material effectivelyOptimizationinfluences the performance measures.Artificial neural network© 2008 Elsevier B.V. All rights reserved.Genetic algorithm1.IntroductionThe selection of appropriate machining conditions forminimum surface roughness during the electric dischargemachining (EDM) process is based on the analysis relating the various process parameters to surface roughness(SR). Traditionally this is carried out by relying heavily onthe operator’s experience or conservative technological dataprovided by the EDM equipment manufacturers, which produced inconsistent machining performance. The parametersettings given by the manufacturers are only applicable forthe common steel grades. The settings for new materials∗Corresponding author. Tel.: +91 9866123121.E-mail address: kmrgurram@rediffmail.com (K.M.R. G.).0924-0136/$ – see front matter © 2008 Elsevier B.V. All rights reserved.doi:10.1016/j.jmatprotec.2008.04.003such as titanium alloys, aluminium alloys, special steels,advanced ceramics and metal matrix composites (MMCs)have to be further optimized experimentally. Optimization ofthe EDM process often proves to be difficult task owing tothe many regulating machining variables. A single parameter change will influence the process in a complex way.Thus the various factors affecting the process have to beunderstood in order to determine the trends of the processvariation. The selection of best combination of the processparameters for an optimal surface roughness involves analytical and statistical methods. In addition, the modeling ofthe process is also an effective way of solving the tediousj o u r n a l o f m a t e r i a l s p r o c e s s i n g t e c h n o l o g y 2 0 9 ( 2 0 0 9 ) 1512–1520NomenclatureAEkImaxIpNQkRaRmaxRmintVWYkZjcurrentsimple mean square errormaximum currentpeak currentnormalized value of the real variablemeasured performancesurface roughnessmaximum values of the real variablesminimum values of the real variablesmachining timeaverage voltageweights of the networkoutput of the networkoutput at the hidden layerproblem of relating the process parameters to the surfaceroughness.The settings for new materials such as titanium alloys,aluminium alloys and special steels have to be further optimized experimentally. It is also aimed to select appropriatemachining conditions for the EDM process based on the analysis relating the various process parameters to SR. It is aimedto develop a methodology using an input–output pattern ofdata from an EDM process to solve both the modeling andoptimization problems. The main objective of this research isto model EDM process for optimum operation representing aparticular problem in the manufacturing environment where,it is not possible to define the optimization objective function using a smooth and continuous mathematical formula.It has been hard to establish models that accurately correlate the process variables and performance of EDM process.Impr ...
Nội dung trích xuất từ tài liệu:
Development of hybrid model and optimization of surface roughness in electric discharge machining using artificial neural networks and genetic algorithmj o u r n a l o f m a t e r i a l s p r o c e s s i n g t e c h n o l o g y 2 0 9 ( 2 0 0 9 ) 1512–1520journal homepage: www.elsevier.com/locate/jmatprotecDevelopment of hybrid model and optimization of surfaceroughness in electric discharge machining using artificialneural networks and genetic algorithmG. Krishna Mohana Rao a,∗ , G. Rangajanardhaa b ,D. Hanumantha Rao c , M. Sreenivasa Rao aabcJNTU College of Engineering, Hyderabad 85, AP, IndiaDepartment of Mechanical Engineering, Hoseo University, South KoreaDeccan College of Engineering and Technology, Hyderabad, AP, Indiaa r t i c l ei n f oa b s t r a c tArticle history:The present work is aimed at optimizing the surface roughness of die sinking electric dis-Received 27 August 2007charge machining (EDM) by considering the simultaneous affect of various input parameters.Received in revised formThe experiments are carried out on Ti6Al4V, HE15, 15CDV6 and M-250. Experiments were28 March 2008conducted by varying the peak current and voltage and the corresponding values of surfaceAccepted 2 April 2008roughness (SR) were measured. Multiperceptron neural network models were developedusing Neuro Solutions package. Genetic algorithm concept is used to optimize the weighting factors of the network. It is observed that the developed model is within the limits of theKeywords:agreeable error when experimental and network model results are compared. It is furtherEDMobserved that the error when the network is optimized by genetic algorithm has come downSurface roughnessto less than 2% from more than 5%. Sensitivity analysis is also done to find the relative influ-Hybrid modelence of factors on the performance measures. It is observed that type of material effectivelyOptimizationinfluences the performance measures.Artificial neural network© 2008 Elsevier B.V. All rights reserved.Genetic algorithm1.IntroductionThe selection of appropriate machining conditions forminimum surface roughness during the electric dischargemachining (EDM) process is based on the analysis relating the various process parameters to surface roughness(SR). Traditionally this is carried out by relying heavily onthe operator’s experience or conservative technological dataprovided by the EDM equipment manufacturers, which produced inconsistent machining performance. The parametersettings given by the manufacturers are only applicable forthe common steel grades. The settings for new materials∗Corresponding author. Tel.: +91 9866123121.E-mail address: kmrgurram@rediffmail.com (K.M.R. G.).0924-0136/$ – see front matter © 2008 Elsevier B.V. All rights reserved.doi:10.1016/j.jmatprotec.2008.04.003such as titanium alloys, aluminium alloys, special steels,advanced ceramics and metal matrix composites (MMCs)have to be further optimized experimentally. Optimization ofthe EDM process often proves to be difficult task owing tothe many regulating machining variables. A single parameter change will influence the process in a complex way.Thus the various factors affecting the process have to beunderstood in order to determine the trends of the processvariation. The selection of best combination of the processparameters for an optimal surface roughness involves analytical and statistical methods. In addition, the modeling ofthe process is also an effective way of solving the tediousj o u r n a l o f m a t e r i a l s p r o c e s s i n g t e c h n o l o g y 2 0 9 ( 2 0 0 9 ) 1512–1520NomenclatureAEkImaxIpNQkRaRmaxRmintVWYkZjcurrentsimple mean square errormaximum currentpeak currentnormalized value of the real variablemeasured performancesurface roughnessmaximum values of the real variablesminimum values of the real variablesmachining timeaverage voltageweights of the networkoutput of the networkoutput at the hidden layerproblem of relating the process parameters to the surfaceroughness.The settings for new materials such as titanium alloys,aluminium alloys and special steels have to be further optimized experimentally. It is also aimed to select appropriatemachining conditions for the EDM process based on the analysis relating the various process parameters to SR. It is aimedto develop a methodology using an input–output pattern ofdata from an EDM process to solve both the modeling andoptimization problems. The main objective of this research isto model EDM process for optimum operation representing aparticular problem in the manufacturing environment where,it is not possible to define the optimization objective function using a smooth and continuous mathematical formula.It has been hard to establish models that accurately correlate the process variables and performance of EDM process.Impr ...
Tìm kiếm theo từ khóa liên quan:
Electro Discharge Machining Surface roughness Hybrid model Optimization Artificial neural network Genetic algorithmTài liệu liên quan:
-
Short-term load forecasting using long short-term memory network
4 trang 50 0 0 -
Applications of artificial neural network in textiles
10 trang 33 0 0 -
Bài giảng Nhập môn Học máy và Khai phá dữ liệu: Chương 8 - Nguyễn Nhật Quang
69 trang 32 0 0 -
Artificial intelligence approach to predict the dynamic modulus of asphalt concrete mixtures
10 trang 31 0 0 -
Phân tích tính hội tụ của thuật toán di truyền lai mới
8 trang 29 0 0 -
8 trang 28 0 0
-
10 trang 27 0 0
-
Ebook Sustainable construction and building materials: Select proceedings of ICSCBM 2018 - Part 2
446 trang 26 0 0 -
Efficient optimization of pump scheduling for reduction of energy costs and greenhouse gas emission
6 trang 25 0 0 -
9 trang 25 0 0