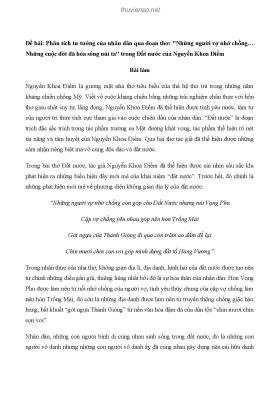
Ebook Applied digital signal processing - Theory and practic: Part 2
Số trang: 559
Loại file: pdf
Dung lượng: 4.81 MB
Lượt xem: 18
Lượt tải: 0
Xem trước 10 trang đầu tiên của tài liệu này:
Thông tin tài liệu:
Part 2 book "Applied digital signal processing - Theory and practic" includes content: Computation of the Discrete Fourier Transform; structures for discrete-time systems, design of FIR filters, design of IIR filters, multirate signal processing.
Nội dung trích xuất từ tài liệu:
Ebook Applied digital signal processing - Theory and practic: Part 2 Computation of the Discrete Fourier 8 Transform This chapter is primarily concerned with algorithms for efficient computation of the Discrete Fourier Transform (DFT). This is an important topic because the DFT plays an important role in the analysis, design, and implementation of many digital signal processing systems. Direct computation of the N -point DFT requires computational cost proportional to N 2 . The most important class of efficient DFT algorithms, known collectively as Fast Fourier Transform (FFT) algorithms, compute all DFT coefficients as a “block” with computational cost proportional to N log2 N . However, when we only need a few DFT coefficients, a few samples of DTFT, or a few values of z -transform, it may be more efficient to use algorithms based on linear filtering operations, like the Goertzel algorithm or the chirp z -transform algorithm. Although many computational environments provide FFT algorithms as built-in func- tions, the user should understand the fundamental principles of FFT algorithms to make effective use of these functions. The details of FFT algorithms are important to designers of real-time DSP systems in either software or hardware. Study objectives After studying this chapter you should be able to: • Understand the derivation, operation, programming, and use of decimation-in-time and decimation-in-frequency radix-2 FFT algorithms. • Understand the general principles underlying the development of FFT algorithms and use them to make effective use of existing functions, evaluate competing algorithms, or guide the selection of algorithms for a particular application or computer architecture. 435 8.1 Direct computation of the Discrete Fourier Transform 8.1 Direct computation of the Discrete Fourier Transform •••••••••••••••••••••••••••••••••••••••••••••••••••••••••••••••••••••••••••••••••••••••••••••••••••••• The DFT of a finite-length sequence of length N is defined by (see Chapter 7) N−1 X[k] = kn x[n]WN , k = 0, 1, . . . , N − 1 (8.1) n=0 2π where WN = e− j N , the root-of-unity, is also known as the twiddle factor. The inverse DFT is given by N−1 1 −kn x[n] = X[k]WN . n = 0, 1, . . . , N − 1 (8.2) N k=0 The two equations differ only in the sign of the exponent of WN and the scaling factor 1/N. Therefore, algorithms developed for computation of the DFT can easily be modified for computation of the inverse DFT. For example, the inverse DFT (8.2) can be written as N−1 ∗ 1 ∗ x[n] = X [k]WN kn . n = 0, 1, . . . , N − 1 (8.3) N k=0 Therefore, the inverse DFT of X[k] can be evaluated by scaling by 1/N the complex conjugate of the DFT of X ∗ [k]; two additional approaches are discussed in Problems 16 and 33. If we use a computer language which supports complex arithmetic we can directly eval- uate formula (8.1) using a double loop. If only real arithmetic is supported by the compiler, we use the formulas N−1 2π 2π XR [k] = xR [n] cos kn + xI [n] sin kn , (8.4a) N N n=0 N−1 2π 2π XI [k] = − xR [n] sin kn − xI [n] cos kn . (8.4b) N N n=0 The DFT coefficients X[k] in (8.1), like any matrix by vector product, can be evaluated using a double loop as shown by the M ATLAB function dftdirect in Figure 8.1. The total cost of computing all X[k] coefficients is approximately N 2 operations when we define an operation to be the work required to execute the statement S=S+W(k,n)*x(n). More specifically, we need to fetch W(k,n), x(n), and the “old” value of S from memory; com- pute the product W(k,n)*x(n) and the sum S+W(k,n)*x(n); and store the result as the “new” value of S. The cost for initialization and other overhead operations is negligible for large values of N and hence will be ignored. We will say that the computational com- plexity of the direct DFT algorithm is “of the order of N 2 ” or O(N 2 ) operations for short. kn For a DFT of fixed size N the values of coefficients WN are usually computed and stored 436 Computation of the Discrete Fourier Transform function Xdft=dftdirect(x) % Direct computation of the DFT N=length(x); Q=2*pi/N; for k=1:N S=0; ...
Nội dung trích xuất từ tài liệu:
Ebook Applied digital signal processing - Theory and practic: Part 2 Computation of the Discrete Fourier 8 Transform This chapter is primarily concerned with algorithms for efficient computation of the Discrete Fourier Transform (DFT). This is an important topic because the DFT plays an important role in the analysis, design, and implementation of many digital signal processing systems. Direct computation of the N -point DFT requires computational cost proportional to N 2 . The most important class of efficient DFT algorithms, known collectively as Fast Fourier Transform (FFT) algorithms, compute all DFT coefficients as a “block” with computational cost proportional to N log2 N . However, when we only need a few DFT coefficients, a few samples of DTFT, or a few values of z -transform, it may be more efficient to use algorithms based on linear filtering operations, like the Goertzel algorithm or the chirp z -transform algorithm. Although many computational environments provide FFT algorithms as built-in func- tions, the user should understand the fundamental principles of FFT algorithms to make effective use of these functions. The details of FFT algorithms are important to designers of real-time DSP systems in either software or hardware. Study objectives After studying this chapter you should be able to: • Understand the derivation, operation, programming, and use of decimation-in-time and decimation-in-frequency radix-2 FFT algorithms. • Understand the general principles underlying the development of FFT algorithms and use them to make effective use of existing functions, evaluate competing algorithms, or guide the selection of algorithms for a particular application or computer architecture. 435 8.1 Direct computation of the Discrete Fourier Transform 8.1 Direct computation of the Discrete Fourier Transform •••••••••••••••••••••••••••••••••••••••••••••••••••••••••••••••••••••••••••••••••••••••••••••••••••••• The DFT of a finite-length sequence of length N is defined by (see Chapter 7) N−1 X[k] = kn x[n]WN , k = 0, 1, . . . , N − 1 (8.1) n=0 2π where WN = e− j N , the root-of-unity, is also known as the twiddle factor. The inverse DFT is given by N−1 1 −kn x[n] = X[k]WN . n = 0, 1, . . . , N − 1 (8.2) N k=0 The two equations differ only in the sign of the exponent of WN and the scaling factor 1/N. Therefore, algorithms developed for computation of the DFT can easily be modified for computation of the inverse DFT. For example, the inverse DFT (8.2) can be written as N−1 ∗ 1 ∗ x[n] = X [k]WN kn . n = 0, 1, . . . , N − 1 (8.3) N k=0 Therefore, the inverse DFT of X[k] can be evaluated by scaling by 1/N the complex conjugate of the DFT of X ∗ [k]; two additional approaches are discussed in Problems 16 and 33. If we use a computer language which supports complex arithmetic we can directly eval- uate formula (8.1) using a double loop. If only real arithmetic is supported by the compiler, we use the formulas N−1 2π 2π XR [k] = xR [n] cos kn + xI [n] sin kn , (8.4a) N N n=0 N−1 2π 2π XI [k] = − xR [n] sin kn − xI [n] cos kn . (8.4b) N N n=0 The DFT coefficients X[k] in (8.1), like any matrix by vector product, can be evaluated using a double loop as shown by the M ATLAB function dftdirect in Figure 8.1. The total cost of computing all X[k] coefficients is approximately N 2 operations when we define an operation to be the work required to execute the statement S=S+W(k,n)*x(n). More specifically, we need to fetch W(k,n), x(n), and the “old” value of S from memory; com- pute the product W(k,n)*x(n) and the sum S+W(k,n)*x(n); and store the result as the “new” value of S. The cost for initialization and other overhead operations is negligible for large values of N and hence will be ignored. We will say that the computational com- plexity of the direct DFT algorithm is “of the order of N 2 ” or O(N 2 ) operations for short. kn For a DFT of fixed size N the values of coefficients WN are usually computed and stored 436 Computation of the Discrete Fourier Transform function Xdft=dftdirect(x) % Direct computation of the DFT N=length(x); Q=2*pi/N; for k=1:N S=0; ...
Tìm kiếm theo từ khóa liên quan:
Applied digital signal processing Digital signal processing Multirate signal processing Design of IIR filters Design of FIR filtersGợi ý tài liệu liên quan:
-
Giáo trình Xử lý số tín hiệu (Digital signal processing): Phần 1
95 trang 58 1 0 -
Lecture Digital signal processing - Chapter 1: Introduction to DSP
30 trang 37 0 0 -
Ebook Digital systems and applications (2/E)
993 trang 34 0 0 -
Lecture Digital signal processing - Chapter 5: IIR digital filter design
158 trang 33 0 0 -
Lecture Digital signal processing - Chapter 3: Discrete time signals and systems
67 trang 27 0 0 -
Lecture Digital signal processing - Chapter 2: Analog-to-digital conversion
41 trang 26 0 0 -
Lecture Digital signal processing - Chapter 6: FIR digital filter design
78 trang 25 0 0 -
Fast fourier transforms: A tutorial review and a state of the art
51 trang 24 0 0 -
Applied processing digital signal
1009 trang 23 0 0 -
Lecture Digital signal processing - Chapter 4: System structures
29 trang 23 0 0