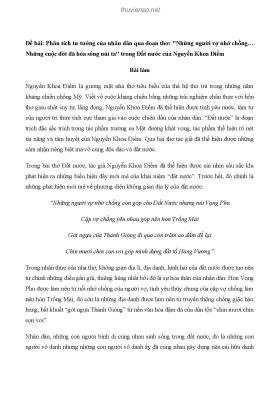
Evaluating effectiveness of ensemble classifiers when detecting fuzzers attacks on the UNSW-NB15 dataset
Số trang: 13
Loại file: pdf
Dung lượng: 299.88 KB
Lượt xem: 6
Lượt tải: 0
Xem trước 2 trang đầu tiên của tài liệu này:
Thông tin tài liệu:
The UNSW-NB15 dataset was created by the Australian Cyber Security Centre in 2015 by using the IXIA tool to extract normal behaviors and modern attacks, it includes normal data and 9 types of attacks with 49 features. Previous research results show that the detection of Fuzzers attacks in this dataset gives the lowest classification quality. This paper analyzes and evaluates the performance of using known ensemble techniques such as Bagging, AdaBoost, Stacking, Decorate, Random Forest and Voting to detect FUZZERS attacks on UNSW-NB15 dataset to create models. The experimental results show that the AdaBoost technique with the component classifiers using decision tree for the best classification quality with F − Measure is 96.76% compared to 94.16%, which is the best result by using single classifiers and 96.36% by using the Random Forest technique.
Nội dung trích xuất từ tài liệu:
Evaluating effectiveness of ensemble classifiers when detecting fuzzers attacks on the UNSW-NB15 dataset
Nội dung trích xuất từ tài liệu:
Evaluating effectiveness of ensemble classifiers when detecting fuzzers attacks on the UNSW-NB15 dataset
Tìm kiếm theo từ khóa liên quan:
Machine learning Ensemble classifier UNSW-NB15 dataset Detecting fuzzers attacks Evaluating effectiveness of ensemble classifiersGợi ý tài liệu liên quan:
-
9 trang 187 0 0
-
Nuclear energy system's behavior and decision making using machine learning
8 trang 111 0 0 -
95 trang 66 0 0
-
Ebook Disrupting finance: FinTech and strategy in the 21st century
194 trang 53 0 0 -
Bài giảng Trí tuệ nhân tạo dành cho mọi người - ThS. Nguyễn Ngọc Tú
149 trang 51 0 0 -
Knowledge management system building blocks
12 trang 46 0 0 -
Ebook The data science design manual: Part 2
240 trang 42 0 0 -
Pseudorandom sequences classification algorithm
8 trang 41 0 0 -
Building credit scoring process in Vietnamese commercial banks using machine learning
14 trang 40 0 0 -
Phát hiện tin giả với python và machine learning
14 trang 39 0 0