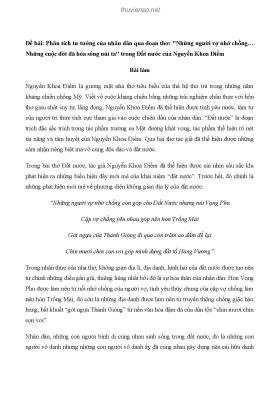
Lecture note Data visualization - Chapter 18
Số trang: 33
Loại file: pptx
Dung lượng: 1.57 MB
Lượt xem: 6
Lượt tải: 0
Xem trước 4 trang đầu tiên của tài liệu này:
Thông tin tài liệu:
This chapter presents the following content: Running time of function, running time of cubic function, running time of quadratic function, running time of linear function, running time of logarithmic function, logarithm, static searching problem,...
Nội dung trích xuất từ tài liệu:
Lecture note Data visualization - Chapter 18Lecture18RecapRunningTimeofFunction RunningTimeofCubicFunction RunningTimeofQuadraticFunction RunningTimeofLinearFunction RunningTimeofLogarithmicFunctionLogarithm BitsinBinaryNumbers RepeatedDoubling RepeatedHalvingCheckinganAlgorithmAnalysisOncewehaveperformedanalgorithmanalysis,wewant todeterminewhetheritiscorrectandasgoodwecan possiblymakeitOnewaytodosoistocodetheprogramandseeifthe empiricallyobservedrunningtimematchestherunning timepredictedbytheanalysisContinued….WhenNincreasesbyafactorof10,therunningtimegoes upbyafactorof10forlinearprograms,100forquadratic programs,and1000forcubicprogramsProgramsthatruninO(NlogN)takeslightlymorethan 10timesaslongtorununderthesamecircumstancesTheseincreasescanbehardtospotifthelowerorder termshaverelativelylargecoefficientsandNisnotlarge enoughAnexampleisthejumpfromN=10toN=100inthe runningtimeforthevariousimplementationsofthe maximumcontiguoussubsequencesumproblemContinued….Anothercommonlyusedtricktoverifythatsomeprogram isO(F(N))istocomputethevaluesT(N)/F(N)forarange ofN,whereT(N)istheempiricallyobservedrunningtimeIfF(N)isatightanswerfortherunningtime,the computedvaluesconvergetoapositiveconstantIfF(N)isanoverestimate,thevaluesconvergetozeroIfF(N)isanunderestimate,andhencewrong,thevalues divergeExampleEmpiricalrunningtimeforNbinarysearchesinanNitemarrayContinued….Noteinparticularthatwedonothavedefinitive convergenceOneproblemisthattheclockthatweusedtotimethe programticksonlyevery10msNotealsothatthereisnogreatdifferencebetweenO(N) andO(NlogN)CertainlyanO(NlogN)algorithmismuchcloserto beinglinearthanbeingquadraticFinally,notethatthemachineinthisexamplehasenough memorytostore640,000objectsLimitationsofBigOhAnalysisBigOhanalysisisaveryeffectivetool,butitdoeshave limitationsItsuseisnotappropriateforsmallamountsofinputForsmallamountsofinput,usethesimplestalgorithmAlso,foraparticularalgorithm,theconstantimpliedby theBigOhmaybetoolargetobepractical Forexample:ifonealgorithmsrunningtimeisgoverned bytheformula2NlogNandanotherhasarunningtimeof 1000N,thefirstalgorithmwouldmostlikelybebetter,even thoughitsgrowthrateislargerContinued….Largeconstantscancomeintoplaywhenanalgorithmis excessivelycomplexTheyalsocomeintoplaybecauseouranalysisdisregards constantsandthuscannotdifferentiatebetweenthingslike memoryaccessanddiskaccessOuranalysisassumesinfinitememory,butinapplications involvinglargedatasets,lackofsufficientmemorycanbe asevereproblemContinued….Sometimes,evenwhenconstantsandlowerorderterms areconsidered,theanalysisisshownempiricallytobean overestimateInthiscase,theanalysisneedstobetightened.Orthe averagecaserunningtimeboundmaybesignificantlyless thantheworstcaserunningtimebound,andsono improvementintheboundispossibleFormanycomplicatedalgorithmstheworstcaseboundis achievablebysomebadinput,butinpracticeitisusually anoverestimateTwoexamples:Continued….However,worstcaseboundsareusuallyeasiertoobtain thantheiraveragecasecounterpartsForexample:amathematicalanalysisoftheaveragecase runningtimeofShellsorthasnotbeenobtainedSometimes,merelydefiningwhataveragemeansis difficultWeuseaworstcaseanalysisbecauseitisexpedientand alsobecause,inmostinstances,theworstcaseanalysisis verymeaningfulInthecourseofperformingtheanalysis,wefrequentlySummaryofChapterInthischapterweintroducedalgorithmanalysisand showedthatalgorithmicdecisionsgenerallyinfluencethe runningtimeofaprogrammuchmorethanprogramming tricksdoWealsoshowedthehugedifferencebetweentherunning timesforquadraticandlinearprogramsandillustratedthat cubicalgorithmsare,forthemostpart,unsatisfactoryWeexaminedanalgorithmthatcouldbeviewedasthe basisforourfirstdatastructureThebinarysearchefficientlysupportsstaticoperations therebyprovidingalogarithmicworstcasesearchCommonErrorsContinued…. DataStructuresandAlgorithmswithMatlabMATLABEnvironmentMATLABopeningwindowMATLABWindowsCommandwindowCommandHistoryWorkspaceWindowCurrentFolderWindowDocumentWindowGraphicsWindowEditWindowStartButtonCommandWindowThecommandwindowislocatedinthecenterpaneofthe defaultviewoftheMATLABscreenThecommandwindowoffersanenvironmentsimilartoa scratchpadUsingitallowsyoutosavethevaluesyoucalculate,but notthecommandsusedtogeneratethosevaluesIfyouwanttosavethecommandsequence,youwillneed tousetheeditingwindowtocreateanMfile.Both approachesarevaluable. ...
Nội dung trích xuất từ tài liệu:
Lecture note Data visualization - Chapter 18Lecture18RecapRunningTimeofFunction RunningTimeofCubicFunction RunningTimeofQuadraticFunction RunningTimeofLinearFunction RunningTimeofLogarithmicFunctionLogarithm BitsinBinaryNumbers RepeatedDoubling RepeatedHalvingCheckinganAlgorithmAnalysisOncewehaveperformedanalgorithmanalysis,wewant todeterminewhetheritiscorrectandasgoodwecan possiblymakeitOnewaytodosoistocodetheprogramandseeifthe empiricallyobservedrunningtimematchestherunning timepredictedbytheanalysisContinued….WhenNincreasesbyafactorof10,therunningtimegoes upbyafactorof10forlinearprograms,100forquadratic programs,and1000forcubicprogramsProgramsthatruninO(NlogN)takeslightlymorethan 10timesaslongtorununderthesamecircumstancesTheseincreasescanbehardtospotifthelowerorder termshaverelativelylargecoefficientsandNisnotlarge enoughAnexampleisthejumpfromN=10toN=100inthe runningtimeforthevariousimplementationsofthe maximumcontiguoussubsequencesumproblemContinued….Anothercommonlyusedtricktoverifythatsomeprogram isO(F(N))istocomputethevaluesT(N)/F(N)forarange ofN,whereT(N)istheempiricallyobservedrunningtimeIfF(N)isatightanswerfortherunningtime,the computedvaluesconvergetoapositiveconstantIfF(N)isanoverestimate,thevaluesconvergetozeroIfF(N)isanunderestimate,andhencewrong,thevalues divergeExampleEmpiricalrunningtimeforNbinarysearchesinanNitemarrayContinued….Noteinparticularthatwedonothavedefinitive convergenceOneproblemisthattheclockthatweusedtotimethe programticksonlyevery10msNotealsothatthereisnogreatdifferencebetweenO(N) andO(NlogN)CertainlyanO(NlogN)algorithmismuchcloserto beinglinearthanbeingquadraticFinally,notethatthemachineinthisexamplehasenough memorytostore640,000objectsLimitationsofBigOhAnalysisBigOhanalysisisaveryeffectivetool,butitdoeshave limitationsItsuseisnotappropriateforsmallamountsofinputForsmallamountsofinput,usethesimplestalgorithmAlso,foraparticularalgorithm,theconstantimpliedby theBigOhmaybetoolargetobepractical Forexample:ifonealgorithmsrunningtimeisgoverned bytheformula2NlogNandanotherhasarunningtimeof 1000N,thefirstalgorithmwouldmostlikelybebetter,even thoughitsgrowthrateislargerContinued….Largeconstantscancomeintoplaywhenanalgorithmis excessivelycomplexTheyalsocomeintoplaybecauseouranalysisdisregards constantsandthuscannotdifferentiatebetweenthingslike memoryaccessanddiskaccessOuranalysisassumesinfinitememory,butinapplications involvinglargedatasets,lackofsufficientmemorycanbe asevereproblemContinued….Sometimes,evenwhenconstantsandlowerorderterms areconsidered,theanalysisisshownempiricallytobean overestimateInthiscase,theanalysisneedstobetightened.Orthe averagecaserunningtimeboundmaybesignificantlyless thantheworstcaserunningtimebound,andsono improvementintheboundispossibleFormanycomplicatedalgorithmstheworstcaseboundis achievablebysomebadinput,butinpracticeitisusually anoverestimateTwoexamples:Continued….However,worstcaseboundsareusuallyeasiertoobtain thantheiraveragecasecounterpartsForexample:amathematicalanalysisoftheaveragecase runningtimeofShellsorthasnotbeenobtainedSometimes,merelydefiningwhataveragemeansis difficultWeuseaworstcaseanalysisbecauseitisexpedientand alsobecause,inmostinstances,theworstcaseanalysisis verymeaningfulInthecourseofperformingtheanalysis,wefrequentlySummaryofChapterInthischapterweintroducedalgorithmanalysisand showedthatalgorithmicdecisionsgenerallyinfluencethe runningtimeofaprogrammuchmorethanprogramming tricksdoWealsoshowedthehugedifferencebetweentherunning timesforquadraticandlinearprogramsandillustratedthat cubicalgorithmsare,forthemostpart,unsatisfactoryWeexaminedanalgorithmthatcouldbeviewedasthe basisforourfirstdatastructureThebinarysearchefficientlysupportsstaticoperations therebyprovidingalogarithmicworstcasesearchCommonErrorsContinued…. DataStructuresandAlgorithmswithMatlabMATLABEnvironmentMATLABopeningwindowMATLABWindowsCommandwindowCommandHistoryWorkspaceWindowCurrentFolderWindowDocumentWindowGraphicsWindowEditWindowStartButtonCommandWindowThecommandwindowislocatedinthecenterpaneofthe defaultviewoftheMATLABscreenThecommandwindowoffersanenvironmentsimilartoa scratchpadUsingitallowsyoutosavethevaluesyoucalculate,but notthecommandsusedtogeneratethosevaluesIfyouwanttosavethecommandsequence,youwillneed tousetheeditingwindowtocreateanMfile.Both approachesarevaluable. ...
Tìm kiếm theo từ khóa liên quan:
Data visualization Lecture note Data visualization Data structures Data Visualization with C Data visualization with matlab Effective graphical displayTài liệu liên quan:
-
Trực quan hóa dữ liệu: Vai trò & thử thách
10 trang 37 0 0 -
Ebook Eloquent JavaScript - A modern introduction to programming: Part 1
199 trang 36 0 0 -
Ebook Management support systems: Part 2 - Dr. Kamlesh Lakhwani
136 trang 32 0 0 -
Lecture Introduction to computing systems (2/e): Chapter 19 - Yale N. Patt, Sanjay J. Patel
28 trang 31 0 0 -
Ebook Introduction to algorithms (3rd edition)
1313 trang 30 0 0 -
Lecture Data Structures: Lesson 38
71 trang 28 0 0 -
Lecture Data structures and algorithms: Chapter 1 - Introduction
41 trang 28 0 0 -
Ebook Introduction to algorithms (Second Edition)
429 trang 27 0 0 -
335 trang 26 0 0
-
Lecture Data Structures: Lesson 32
16 trang 25 0 0