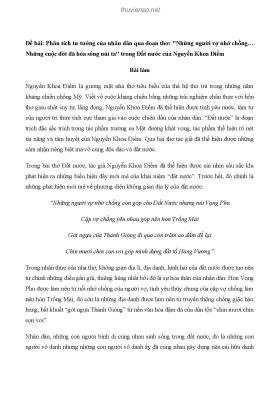
Bài giảng Tối ưu hóa nâng cao: Chương 5 - Hoàng Nam Dũng
Số trang: 31
Loại file: pdf
Dung lượng: 1.26 MB
Lượt xem: 10
Lượt tải: 0
Xem trước 4 trang đầu tiên của tài liệu này:
Thông tin tài liệu:
Bài giảng "Tối ưu hóa nâng cao - Chương 5: Gradient descent" cung cấp cho người học các kiến thức: Gradient descent, gradient descent interpretation, fixed step size, backtracking line search,... Mời các bạn cùng tham khảo.
Nội dung trích xuất từ tài liệu:
Bài giảng Tối ưu hóa nâng cao: Chương 5 - Hoàng Nam DũngGradient DescentHoàng Nam DũngKhoa Toán - Cơ - Tin học, Đại học Khoa học Tự nhiên, Đại học Quốc gia Hà NộiGradient descent Consider unconstrained, smooth convex optimization min f (x) x with convex and differentiable function f : Rn → R. Denote the optimal value by f ∗ = minx f (x) and a solution by x ∗ . 1Gradient descent Consider unconstrained, smooth convex optimization min f (x) x with convex and differentiable function f : Rn → R. Denote the optimal value by f ∗ = minx f (x) and a solution by x ∗ . Gradient descent: choose initial point x (0) ∈ Rn , repeat: x (k) = x (k−1) − tk · ∇f (x (k−1) ), k = 1, 2, 3, . . . Stop at some point. 1● ● ● ● ● 4 2● ●●●● 53Gradient descent interpretation At each iteration, consider the expansion 1 2 f (y ) ≈ f (x) + ∇f (x)T (y − x) + ky − xk2 . 2t Quadratic approximation, replacing usual Hessian ∇2 f (x) by 1t I . f (x) + ∇f (x)T (y − x) linear approximation to f 1 2 2t ky − xk 2 proximity term to x, with weight 1/2t Choose next point y = x + to minimize quadratic approximation x + = x − t∇f (x). 4Gradient descent interpretation ● ● Blue point Blue pointisisx,x, redred point is is point ∗ T x = argminy f (x) + ∇f (x) (y − x) + 1 ky −1 xk22 + T ky − xk22 2t x = argmin f (x) + ∇f (x) (y − x) + y 2t 5Outline I How to choose step sizes I Convergence analysis I Nonconvex functions I Gradient boosting 6Fixed step size Fixed step size Simply take ttkk ==t tfor Simply take forallallk k==1,1, 3, .3,. .,. .can 2, 2, diverge ., can if t is diverge if too t is big. too big. 2 2 2 2 Consider f (x) = (10x Consider f (x) = (10x + x )/2, gradient descent after 1 1 +2 x2 )/2, gradient descent after 8 steps: 8 steps: 20 ● 10 ● ● * 0 −10 −20 −20 −10 0 10 20 7 9Fixed step size Can be Can be slow slow ifif tt isis too toosmall. small.Same example, Same gradient example, descent gradient after after descent 100 steps: 100 steps: 20 ...
Nội dung trích xuất từ tài liệu:
Bài giảng Tối ưu hóa nâng cao: Chương 5 - Hoàng Nam DũngGradient DescentHoàng Nam DũngKhoa Toán - Cơ - Tin học, Đại học Khoa học Tự nhiên, Đại học Quốc gia Hà NộiGradient descent Consider unconstrained, smooth convex optimization min f (x) x with convex and differentiable function f : Rn → R. Denote the optimal value by f ∗ = minx f (x) and a solution by x ∗ . 1Gradient descent Consider unconstrained, smooth convex optimization min f (x) x with convex and differentiable function f : Rn → R. Denote the optimal value by f ∗ = minx f (x) and a solution by x ∗ . Gradient descent: choose initial point x (0) ∈ Rn , repeat: x (k) = x (k−1) − tk · ∇f (x (k−1) ), k = 1, 2, 3, . . . Stop at some point. 1● ● ● ● ● 4 2● ●●●● 53Gradient descent interpretation At each iteration, consider the expansion 1 2 f (y ) ≈ f (x) + ∇f (x)T (y − x) + ky − xk2 . 2t Quadratic approximation, replacing usual Hessian ∇2 f (x) by 1t I . f (x) + ∇f (x)T (y − x) linear approximation to f 1 2 2t ky − xk 2 proximity term to x, with weight 1/2t Choose next point y = x + to minimize quadratic approximation x + = x − t∇f (x). 4Gradient descent interpretation ● ● Blue point Blue pointisisx,x, redred point is is point ∗ T x = argminy f (x) + ∇f (x) (y − x) + 1 ky −1 xk22 + T ky − xk22 2t x = argmin f (x) + ∇f (x) (y − x) + y 2t 5Outline I How to choose step sizes I Convergence analysis I Nonconvex functions I Gradient boosting 6Fixed step size Fixed step size Simply take ttkk ==t tfor Simply take forallallk k==1,1, 3, .3,. .,. .can 2, 2, diverge ., can if t is diverge if too t is big. too big. 2 2 2 2 Consider f (x) = (10x Consider f (x) = (10x + x )/2, gradient descent after 1 1 +2 x2 )/2, gradient descent after 8 steps: 8 steps: 20 ● 10 ● ● * 0 −10 −20 −20 −10 0 10 20 7 9Fixed step size Can be Can be slow slow ifif tt isis too toosmall. small.Same example, Same gradient example, descent gradient after after descent 100 steps: 100 steps: 20 ...
Tìm kiếm theo từ khóa liên quan:
Bài giảng Tối ưu hóa nâng cao Tối ưu hóa nâng cao Tối ưu hóa Gradient descent Fixed step sizeTài liệu liên quan:
-
Tóm tắt luận án tiến sỹ Một số vấn đề tối ưu hóa và nâng cao hiệu quả trong xử lý thông tin hình ảnh
28 trang 225 0 0 -
BÀI TẬP TỔNG HỢP - QUY HOẠCH TUYẾN TÍNH
3 trang 69 0 0 -
Giáo trình Tối ưu hóa - PGS.TS. Nguyễn Hải Thanh
187 trang 45 0 0 -
Tổng hợp bài tập Tối ưu hoá: Phần 2
152 trang 36 0 0 -
Giáo trình tối ưu hóa - Chương 5
31 trang 35 0 0 -
Tổng hợp bài tập Tối ưu hoá: Phần 1
177 trang 29 0 0 -
Bài giảng Lý thuyết tối ưu - Phan Lê Na
181 trang 29 0 0 -
Công nghiệp thực phẩm và quá trình tối ưu hóa: Phần 1
174 trang 29 0 0 -
7 trang 29 0 0
-
Giáo trình tối ưu hóa - Chương 2
28 trang 28 0 0