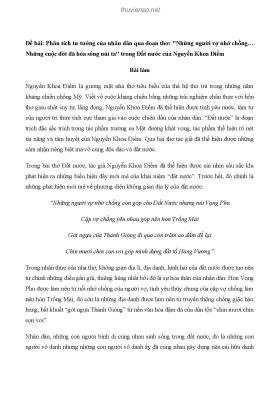
Bài giảng Tối ưu hóa nâng cao: Chương 6 - Hoàng Nam Dũng
Số trang: 36
Loại file: pdf
Dung lượng: 747.96 KB
Lượt xem: 9
Lượt tải: 0
Xem trước 4 trang đầu tiên của tài liệu này:
Thông tin tài liệu:
Bài giảng "Tối ưu hóa nâng cao - Chương 6: Subgradients" cung cấp cho người học các kiến thức: Last time - gradient descent, subgradients, examples of subgradients, monotonicity, examples of non-subdifferentiable functions,... Mời các bạn cùng tham khảo.
Nội dung trích xuất từ tài liệu:
Bài giảng Tối ưu hóa nâng cao: Chương 6 - Hoàng Nam DũngSubgradientsHoàng Nam DũngKhoa Toán - Cơ - Tin học, Đại học Khoa học Tự nhiên, Đại học Quốc gia Hà NộiLast time: gradient descent Consider the problem min f (x) x for f convex and differentiable, dom(f ) = Rn . Gradient descent: choose initial x (0) ∈ Rn , repeat x (k) = x (k−1) − tk · ∇f (x (k−1) ), k = 1, 2, 3, . . . Step sizes tk chosen to be fixed and small, or by backtracking line search If ∇f Lipschitz, gradient descent has convergence rate O(1/ε) Downsides: I Requires f differentiable I Can be slow to converge 1Outline Today: I Subgradients I Examples I Properties I Optimality characterizations 2Basic inequality Basic inequality recall the basic inequality for differentiable convex functions: Recall that for convex and differentiable f , T T f (y )f≥ (y)f (x) + ∇f ≥ f (x) (x)(x) + ∇f −−x), (y (y x) ∀x, ∀yy∈∈dom dom(f f ). (x, f (x)) ∇f (x) −1 I The first-order approximation of f at x is a global lower • the first-order approximation of f at x is a global lower bound bound. • ∇f (x) defines a non-vertical supporting hyperplane to epi f at (x, f (x)): I ∇f defines a non-vertical supporting hyperplane to epi(f ) at T (x, f (x)) ∇f (x) y − x ≤ 0 ∀(y, t) ∈ epi f −1 y t! f (x)!! x ∇f −1 − ≤ 0, ∀(y , t) ∈ epi(f ). Subgradients t f (x) 4-2 3Subgradients A subgradient of a convex function f at x is any g ∈ Rn such that f (y ) ≥ f (x) + g T (y − x), ∀y ∈ dom(f ). I Always exists (on the relative interior of dom(f )) I If f differentiable at x, then g = ∇f (x) uniquely I Same definition works for nonconvex f (however, subgradients need not exist). 4Subgradients A subgradient of a convex function f at x is any g ∈ Rn such that f (y ) ≥ f (x) + g T (y − x), ∀y ∈ dom(f ). I Always exists (on the relative interior of dom(f )) Subgradient I If f differentiable at x, then g = ∇f (x) uniquely g is a subgradient of a convex function f at x ∈ dom f if I Same definition works for nonconvex f (however, subgradients need not exist).f (y) ≥ f (x) + gT (y − x) ∀y ∈ dom f f (y) f (x1) + g1T (y − x1) f (x1) + g2T (y − x1) f (x2) + g3T (y − x2) x1 x2 g1 and g2 are subgradients at x1 , g3 is subgradient at x2 . 4 g1, g2 are subgradients at x1; g3 is a subgradient at x2 Examples of subgradientsExamples of subgradients Considerff :: RR → Consider R, ff (x) = |x| → R, 2.0 1.5 1.0 f(x) 0.5 0.0 −0.5 −2 −1 0 1 2 x • For x 6= 0, unique subgradient g = sign(x) I For x 6= 0, unique subgradient g = sign(x) • For x = 0, subgradient g is any element of [−1, 1] I For x = 0, subgradient g is any element of [−1, 1]. 5 5Examples of subgradients Considerf f: :RRnn→ Consider R,ff (x) →R, (x) = kxk2 = kxk 2 f(x) x2 x1 x Forxx6=6=0,0,unique • IFor subgradientg g==x/kxk2 uniquesubgradient kxk2 • IFor Forxx==0,0,subgradient subgradientg gis isany elementofof{z{z: kzk anyelement : kzk ≤≤1}1}. 2 2 6Examples of subgradients Considerff :: RRnn → Consi ...
Nội dung trích xuất từ tài liệu:
Bài giảng Tối ưu hóa nâng cao: Chương 6 - Hoàng Nam DũngSubgradientsHoàng Nam DũngKhoa Toán - Cơ - Tin học, Đại học Khoa học Tự nhiên, Đại học Quốc gia Hà NộiLast time: gradient descent Consider the problem min f (x) x for f convex and differentiable, dom(f ) = Rn . Gradient descent: choose initial x (0) ∈ Rn , repeat x (k) = x (k−1) − tk · ∇f (x (k−1) ), k = 1, 2, 3, . . . Step sizes tk chosen to be fixed and small, or by backtracking line search If ∇f Lipschitz, gradient descent has convergence rate O(1/ε) Downsides: I Requires f differentiable I Can be slow to converge 1Outline Today: I Subgradients I Examples I Properties I Optimality characterizations 2Basic inequality Basic inequality recall the basic inequality for differentiable convex functions: Recall that for convex and differentiable f , T T f (y )f≥ (y)f (x) + ∇f ≥ f (x) (x)(x) + ∇f −−x), (y (y x) ∀x, ∀yy∈∈dom dom(f f ). (x, f (x)) ∇f (x) −1 I The first-order approximation of f at x is a global lower • the first-order approximation of f at x is a global lower bound bound. • ∇f (x) defines a non-vertical supporting hyperplane to epi f at (x, f (x)): I ∇f defines a non-vertical supporting hyperplane to epi(f ) at T (x, f (x)) ∇f (x) y − x ≤ 0 ∀(y, t) ∈ epi f −1 y t! f (x)!! x ∇f −1 − ≤ 0, ∀(y , t) ∈ epi(f ). Subgradients t f (x) 4-2 3Subgradients A subgradient of a convex function f at x is any g ∈ Rn such that f (y ) ≥ f (x) + g T (y − x), ∀y ∈ dom(f ). I Always exists (on the relative interior of dom(f )) I If f differentiable at x, then g = ∇f (x) uniquely I Same definition works for nonconvex f (however, subgradients need not exist). 4Subgradients A subgradient of a convex function f at x is any g ∈ Rn such that f (y ) ≥ f (x) + g T (y − x), ∀y ∈ dom(f ). I Always exists (on the relative interior of dom(f )) Subgradient I If f differentiable at x, then g = ∇f (x) uniquely g is a subgradient of a convex function f at x ∈ dom f if I Same definition works for nonconvex f (however, subgradients need not exist).f (y) ≥ f (x) + gT (y − x) ∀y ∈ dom f f (y) f (x1) + g1T (y − x1) f (x1) + g2T (y − x1) f (x2) + g3T (y − x2) x1 x2 g1 and g2 are subgradients at x1 , g3 is subgradient at x2 . 4 g1, g2 are subgradients at x1; g3 is a subgradient at x2 Examples of subgradientsExamples of subgradients Considerff :: RR → Consider R, ff (x) = |x| → R, 2.0 1.5 1.0 f(x) 0.5 0.0 −0.5 −2 −1 0 1 2 x • For x 6= 0, unique subgradient g = sign(x) I For x 6= 0, unique subgradient g = sign(x) • For x = 0, subgradient g is any element of [−1, 1] I For x = 0, subgradient g is any element of [−1, 1]. 5 5Examples of subgradients Considerf f: :RRnn→ Consider R,ff (x) →R, (x) = kxk2 = kxk 2 f(x) x2 x1 x Forxx6=6=0,0,unique • IFor subgradientg g==x/kxk2 uniquesubgradient kxk2 • IFor Forxx==0,0,subgradient subgradientg gis isany elementofof{z{z: kzk anyelement : kzk ≤≤1}1}. 2 2 6Examples of subgradients Considerff :: RRnn → Consi ...
Tìm kiếm theo từ khóa liên quan:
Bài giảng Tối ưu hóa nâng cao Tối ưu hóa nâng cao Tối ưu hóa Gradient descent Subdifferentiable functionsTài liệu liên quan:
-
Tóm tắt luận án tiến sỹ Một số vấn đề tối ưu hóa và nâng cao hiệu quả trong xử lý thông tin hình ảnh
28 trang 225 0 0 -
BÀI TẬP TỔNG HỢP - QUY HOẠCH TUYẾN TÍNH
3 trang 68 0 0 -
Giáo trình Tối ưu hóa - PGS.TS. Nguyễn Hải Thanh
187 trang 45 0 0 -
Tổng hợp bài tập Tối ưu hoá: Phần 2
152 trang 36 0 0 -
Giáo trình tối ưu hóa - Chương 5
31 trang 35 0 0 -
Tổng hợp bài tập Tối ưu hoá: Phần 1
177 trang 29 0 0 -
Bài giảng Lý thuyết tối ưu - Phan Lê Na
181 trang 29 0 0 -
Công nghiệp thực phẩm và quá trình tối ưu hóa: Phần 1
174 trang 29 0 0 -
7 trang 29 0 0
-
Giáo trình tối ưu hóa - Chương 2
28 trang 28 0 0