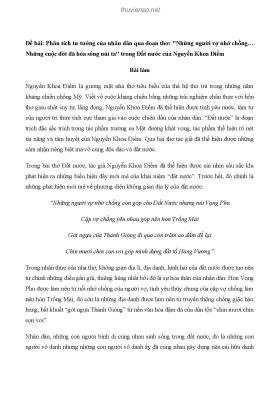
Bài giảng Tối ưu hóa nâng cao: Chương 9 - Hoàng Nam Dũng
Số trang: 24
Loại file: pdf
Dung lượng: 381.80 KB
Lượt xem: 8
Lượt tải: 0
Xem trước 3 trang đầu tiên của tài liệu này:
Thông tin tài liệu:
Bài giảng "Tối ưu hóa nâng cao - Chương 9: Stochastic gradient descent" cung cấp cho người học các kiến thức: Proximal gradient descent, stochastic gradient descent, convergence rates, early stopping, mini-batches. Mời các bạn cùng tham khảo.
Nội dung trích xuất từ tài liệu:
Bài giảng Tối ưu hóa nâng cao: Chương 9 - Hoàng Nam DũngStochastic Gradient DescentHoàng Nam DũngKhoa Toán - Cơ - Tin học, Đại học Khoa học Tự nhiên, Đại học Quốc gia Hà NộiLast time: proximal gradient descent Consider the problem min g (x) + h(x) x with g , h convex, g differentiable, and h “simple” in so much as 1 proxt (x) = argminz kx − zk22 + h(z) 2t is computable. Proximal gradient descent: let x (0) ∈ Rn , repeat: x (k) = proxtk (x (k−1) − tk ∇g (x (k−1) )), k = 1, 2, 3, . . . Step sizes tk chosen to be fixed and small, or via backtracking. If ∇g is Lipschitz with constant L, then this has convergence rate √ O(1/ε). Lastly we can accelerate this, to optimal rate O(1/ ε). 1Outline Today: I Stochastic gradient descent I Convergence rates I Mini-batches I Early stopping 2Stochastic gradient descent Consider minimizing an average of functions m 1 X min fi (x). x m i=1 Pm Pm As ∇ i=1 fi (x) = i=1 ∇fi (x), gradient descent would repeat m 1 X x (k) = x (k−1) − tk · ∇fi (x (k−1) ), k = 1, 2, 3, . . . m i=1 In comparison, stochastic gradient descent or SGD (or incremental gradient descent) repeats: x (k) = x (k−1) − tk · ∇fik (x (k−1) ), k = 1, 2, 3, . . . where ik ∈ {1, . . . , m} is some chosen index at iteration k. 3Stochastic gradient descent Two rules for choosing index ik at iteration k: I Randomized rule: choose ik ∈ {1, ...m} uniformly at random. I Cyclic rule: choose ik = 1, 2, . . . , m, 1, 2, . . . , m, . . . Randomized rule is more common in practice. For randomized rule, note that E[∇fik (x)] = ∇f (x), so we can view SGD as using an unbiased estimate of the gradient at each step. Main appeal of SGD: I Iteration cost is independent of m (number of functions). I Can also be a big savings in terms of memory usage. 4Example: stochastic logistic regression Given (xi , yi ) ∈ Rp × {0, 1}, i = 1, . . . , n, recall logistic regression n 1 X min f (β) = −yi xiT β + log(1 + exp(xiT β)) . β n i=1 | {z } fi (β) 1 Pn Gradient computation ∇f (β) = i=1 (yi − pi (β))xi is doable n when n is moderate, but not when n is huge. Full gradient (also called batch) versus stochastic gradient: I One batch update costs O(np). I One stochastic update costs O(p). Clearly, e.g., 10K stochastic steps are much more affordable. 5Batch vs. stochastic gradient descent Small example with n = 10, p = 2 to show the “classic picture” for Small example with n = 10, p = 2 to show the “classic picture” for batch versus stochastic methods: batch versus stochastic methods: ● Batch Blue: batch steps, O(np) 20 ● Random Red: batch Blue: stochastic steps,steps, O(np)O(p) Red: stochastic steps, O(p) Rule of thumb for stochastic 10 methods: Rule of thumb for stochastic ● ● ● ●● * ●●● ●● methods: I generally thrive far from 0 ● ● ● ●● ● ● ●● ● ● ● ● ● ● ● ● ●● ● ● ● ● ● ●● ●● ●● ● ● ● • generally optimumthrive far ●● ● Ifrom optimum −10 ●●● ● ● ●● ...
Nội dung trích xuất từ tài liệu:
Bài giảng Tối ưu hóa nâng cao: Chương 9 - Hoàng Nam DũngStochastic Gradient DescentHoàng Nam DũngKhoa Toán - Cơ - Tin học, Đại học Khoa học Tự nhiên, Đại học Quốc gia Hà NộiLast time: proximal gradient descent Consider the problem min g (x) + h(x) x with g , h convex, g differentiable, and h “simple” in so much as 1 proxt (x) = argminz kx − zk22 + h(z) 2t is computable. Proximal gradient descent: let x (0) ∈ Rn , repeat: x (k) = proxtk (x (k−1) − tk ∇g (x (k−1) )), k = 1, 2, 3, . . . Step sizes tk chosen to be fixed and small, or via backtracking. If ∇g is Lipschitz with constant L, then this has convergence rate √ O(1/ε). Lastly we can accelerate this, to optimal rate O(1/ ε). 1Outline Today: I Stochastic gradient descent I Convergence rates I Mini-batches I Early stopping 2Stochastic gradient descent Consider minimizing an average of functions m 1 X min fi (x). x m i=1 Pm Pm As ∇ i=1 fi (x) = i=1 ∇fi (x), gradient descent would repeat m 1 X x (k) = x (k−1) − tk · ∇fi (x (k−1) ), k = 1, 2, 3, . . . m i=1 In comparison, stochastic gradient descent or SGD (or incremental gradient descent) repeats: x (k) = x (k−1) − tk · ∇fik (x (k−1) ), k = 1, 2, 3, . . . where ik ∈ {1, . . . , m} is some chosen index at iteration k. 3Stochastic gradient descent Two rules for choosing index ik at iteration k: I Randomized rule: choose ik ∈ {1, ...m} uniformly at random. I Cyclic rule: choose ik = 1, 2, . . . , m, 1, 2, . . . , m, . . . Randomized rule is more common in practice. For randomized rule, note that E[∇fik (x)] = ∇f (x), so we can view SGD as using an unbiased estimate of the gradient at each step. Main appeal of SGD: I Iteration cost is independent of m (number of functions). I Can also be a big savings in terms of memory usage. 4Example: stochastic logistic regression Given (xi , yi ) ∈ Rp × {0, 1}, i = 1, . . . , n, recall logistic regression n 1 X min f (β) = −yi xiT β + log(1 + exp(xiT β)) . β n i=1 | {z } fi (β) 1 Pn Gradient computation ∇f (β) = i=1 (yi − pi (β))xi is doable n when n is moderate, but not when n is huge. Full gradient (also called batch) versus stochastic gradient: I One batch update costs O(np). I One stochastic update costs O(p). Clearly, e.g., 10K stochastic steps are much more affordable. 5Batch vs. stochastic gradient descent Small example with n = 10, p = 2 to show the “classic picture” for Small example with n = 10, p = 2 to show the “classic picture” for batch versus stochastic methods: batch versus stochastic methods: ● Batch Blue: batch steps, O(np) 20 ● Random Red: batch Blue: stochastic steps,steps, O(np)O(p) Red: stochastic steps, O(p) Rule of thumb for stochastic 10 methods: Rule of thumb for stochastic ● ● ● ●● * ●●● ●● methods: I generally thrive far from 0 ● ● ● ●● ● ● ●● ● ● ● ● ● ● ● ● ●● ● ● ● ● ● ●● ●● ●● ● ● ● • generally optimumthrive far ●● ● Ifrom optimum −10 ●●● ● ● ●● ...
Tìm kiếm theo từ khóa liên quan:
Bài giảng Tối ưu hóa nâng cao Tối ưu hóa nâng cao Tối ưu hóa Stochastic gradient descent Convergence rates Early stoppingTài liệu liên quan:
-
Tóm tắt luận án tiến sỹ Một số vấn đề tối ưu hóa và nâng cao hiệu quả trong xử lý thông tin hình ảnh
28 trang 225 0 0 -
BÀI TẬP TỔNG HỢP - QUY HOẠCH TUYẾN TÍNH
3 trang 68 0 0 -
Giáo trình Tối ưu hóa - PGS.TS. Nguyễn Hải Thanh
187 trang 45 0 0 -
Tổng hợp bài tập Tối ưu hoá: Phần 2
152 trang 36 0 0 -
Giáo trình tối ưu hóa - Chương 5
31 trang 35 0 0 -
Tổng hợp bài tập Tối ưu hoá: Phần 1
177 trang 29 0 0 -
Bài giảng Lý thuyết tối ưu - Phan Lê Na
181 trang 29 0 0 -
Công nghiệp thực phẩm và quá trình tối ưu hóa: Phần 1
174 trang 29 0 0 -
7 trang 29 0 0
-
Giáo trình tối ưu hóa - Chương 2
28 trang 28 0 0