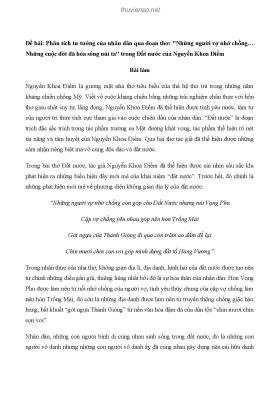
Báo cáo hóa học: Research Article Noisy Sparse Recovery Based on Parameterized Quadratic Programming by Thresholding Jun Zhang,1, 2 Yuanqing Li,1 Zhuliang Yu,1 and
Số trang: 7
Loại file: pdf
Dung lượng: 659.71 KB
Lượt xem: 10
Lượt tải: 0
Xem trước 2 trang đầu tiên của tài liệu này:
Thông tin tài liệu:
Tuyển tập báo cáo các nghiên cứu khoa học quốc tế ngành hóa học dành cho các bạn yêu hóa học tham khảo đề tài: Research Article Noisy Sparse Recovery Based on Parameterized Quadratic Programming by ThresholdingJun Zhang,1, 2 Yuanqing Li,1 Zhuliang Yu,1 and
Nội dung trích xuất từ tài liệu:
Báo cáo hóa học: " Research Article Noisy Sparse Recovery Based on Parameterized Quadratic Programming by Thresholding Jun Zhang,1, 2 Yuanqing Li,1 Zhuliang Yu,1 and "Hindawi Publishing CorporationEURASIP Journal on Advances in Signal ProcessingVolume 2011, Article ID 528734, 7 pagesdoi:10.1155/2011/528734Research ArticleNoisy Sparse Recovery Based on Parameterized QuadraticProgramming by Thresholding Jun Zhang,1, 2 Yuanqing Li,1 Zhuliang Yu,1 and Zhenghui Gu1 1 Center for Brain-Computer Interfaces and Brain Information Processing, College of Automation Science and Engineering, South China University of Technology, Guangzhou 510640, China 2 College of Information Engineering, Guangdong University of Technology, Guangzhou 510006, China Correspondence should be addressed to Jun Zhang, zhangjun7907@hotmail.com Received 27 August 2010; Revised 12 December 2010; Accepted 28 January 2011 Academic Editor: Walter Kellermann Copyright © 2011 Jun Zhang et al. This is an open access article distributed under the Creative Commons Attribution License, which permits unrestricted use, distribution, and reproduction in any medium, provided the original work is properly cited. Parameterized quadratic programming (Lasso) is a powerful tool for the recovery of sparse signals based on underdetermined observations contaminated by noise. In this paper, we study the problem of simultaneous sparsity pattern recovery and approximation recovery based on the Lasso. An extended Lasso method is proposed with the following main contributions: (1) we analyze the recovery accuracy of Lasso under the condition of guaranteeing the recovery of nonzero entries positions. Specifically, an upper bound of the tuning parameter h of Lasso is derived. If h exceeds this bound, the recovery error will increase with h; (2) an extended Lasso algorithm is developed by choosing the tuning parameter according to the bound and at the same time deriving a threshold to recover zero entries from the output of the Lasso. The simulation results validate that our method produces higher probability of sparsity pattern recovery and better approximation recovery compared to two state-of-the-art Lasso methods. Here and throughout, · p denotes the L p -norm (0 ≤1. Introduction p ≤ ∞). Specially, S 0 = | supp(S)|, where supp(S): =The problem of recovering unknown sparse vector S ∈ R m { j | S j = 0}, |Ω| denotes the cardinality of a finite set Ω /based on the limited noisy observations Y = AS + e arises and S j denotes the j th component in the vector S. In thein many applications, including compressed sensing [1, 2], optimization problem (1), the tuning parameter h is criticalpattern recognition [3, 4], blind source separation [5, 6], for deriving a satisfactory solution.signal reconstruction [7], and machine learning [8], where Up to date, many theoretical results have been obtainedA ∈ R n×m is referred to a measurement matrix with n < on Lasso to recover a sparse signal. The following twom and e ∈ R n is an unknown vector of noise. In this scenarios are usually of interest:paper, we suppose the positions and the signs of nonzero (1) Sparsity pattern recovery: given noisy observations Ycomponents of S are distributed uniformly at random, and of sparse signal S, how to recover the positions andtheir amplitudes follow an arbitrary distribution. We also signs of S’s nonzero entries.assume that e follows zero-mean, independent, and identi-cally distributed sub-Gaussian with parameter σ 2 . Recently, (2) Stable recovery: analyzing the error bound betweenmany studies have advocated the use of the parameterized Lasso solution S and true sparse vector S.quadratic programming (Lasso [9, 10], also called basispursuit [11]) to deal with the noisy sparse recovery problem About scenario (1), based on deterministic framework, Fuchs [12, 13] has provided a sufficient condition in mutualthrough minimizing the following objective function whichsimultaneously executes approximation and stable recovery incoherence form. Tropp [14] a ...
Nội dung trích xuất từ tài liệu:
Báo cáo hóa học: " Research Article Noisy Sparse Recovery Based on Parameterized Quadratic Programming by Thresholding Jun Zhang,1, 2 Yuanqing Li,1 Zhuliang Yu,1 and "Hindawi Publishing CorporationEURASIP Journal on Advances in Signal ProcessingVolume 2011, Article ID 528734, 7 pagesdoi:10.1155/2011/528734Research ArticleNoisy Sparse Recovery Based on Parameterized QuadraticProgramming by Thresholding Jun Zhang,1, 2 Yuanqing Li,1 Zhuliang Yu,1 and Zhenghui Gu1 1 Center for Brain-Computer Interfaces and Brain Information Processing, College of Automation Science and Engineering, South China University of Technology, Guangzhou 510640, China 2 College of Information Engineering, Guangdong University of Technology, Guangzhou 510006, China Correspondence should be addressed to Jun Zhang, zhangjun7907@hotmail.com Received 27 August 2010; Revised 12 December 2010; Accepted 28 January 2011 Academic Editor: Walter Kellermann Copyright © 2011 Jun Zhang et al. This is an open access article distributed under the Creative Commons Attribution License, which permits unrestricted use, distribution, and reproduction in any medium, provided the original work is properly cited. Parameterized quadratic programming (Lasso) is a powerful tool for the recovery of sparse signals based on underdetermined observations contaminated by noise. In this paper, we study the problem of simultaneous sparsity pattern recovery and approximation recovery based on the Lasso. An extended Lasso method is proposed with the following main contributions: (1) we analyze the recovery accuracy of Lasso under the condition of guaranteeing the recovery of nonzero entries positions. Specifically, an upper bound of the tuning parameter h of Lasso is derived. If h exceeds this bound, the recovery error will increase with h; (2) an extended Lasso algorithm is developed by choosing the tuning parameter according to the bound and at the same time deriving a threshold to recover zero entries from the output of the Lasso. The simulation results validate that our method produces higher probability of sparsity pattern recovery and better approximation recovery compared to two state-of-the-art Lasso methods. Here and throughout, · p denotes the L p -norm (0 ≤1. Introduction p ≤ ∞). Specially, S 0 = | supp(S)|, where supp(S): =The problem of recovering unknown sparse vector S ∈ R m { j | S j = 0}, |Ω| denotes the cardinality of a finite set Ω /based on the limited noisy observations Y = AS + e arises and S j denotes the j th component in the vector S. In thein many applications, including compressed sensing [1, 2], optimization problem (1), the tuning parameter h is criticalpattern recognition [3, 4], blind source separation [5, 6], for deriving a satisfactory solution.signal reconstruction [7], and machine learning [8], where Up to date, many theoretical results have been obtainedA ∈ R n×m is referred to a measurement matrix with n < on Lasso to recover a sparse signal. The following twom and e ∈ R n is an unknown vector of noise. In this scenarios are usually of interest:paper, we suppose the positions and the signs of nonzero (1) Sparsity pattern recovery: given noisy observations Ycomponents of S are distributed uniformly at random, and of sparse signal S, how to recover the positions andtheir amplitudes follow an arbitrary distribution. We also signs of S’s nonzero entries.assume that e follows zero-mean, independent, and identi-cally distributed sub-Gaussian with parameter σ 2 . Recently, (2) Stable recovery: analyzing the error bound betweenmany studies have advocated the use of the parameterized Lasso solution S and true sparse vector S.quadratic programming (Lasso [9, 10], also called basispursuit [11]) to deal with the noisy sparse recovery problem About scenario (1), based on deterministic framework, Fuchs [12, 13] has provided a sufficient condition in mutualthrough minimizing the following objective function whichsimultaneously executes approximation and stable recovery incoherence form. Tropp [14] a ...
Tìm kiếm theo từ khóa liên quan:
báo cáo khoa học báo cáo hóa học công trình nghiên cứu về hóa học tài liệu về hóa học cách trình bày báo cáoTài liệu liên quan:
-
HƯỚNG DẪN THỰC TẬP VÀ VIẾT BÁO CÁO THỰC TẬP TỐT NGHIỆP
18 trang 358 0 0 -
63 trang 319 0 0
-
13 trang 266 0 0
-
Báo cáo khoa học Bước đầu tìm hiểu văn hóa ẩm thực Trà Vinh
61 trang 254 0 0 -
Hướng dẫn thực tập tốt nghiệp dành cho sinh viên đại học Ngành quản trị kinh doanh
20 trang 237 0 0 -
Tóm tắt luận án tiến sỹ Một số vấn đề tối ưu hóa và nâng cao hiệu quả trong xử lý thông tin hình ảnh
28 trang 224 0 0 -
Đồ án: Nhà máy thủy điện Vĩnh Sơn - Bình Định
54 trang 223 0 0 -
23 trang 211 0 0
-
Đề tài nghiên cứu khoa học và công nghệ cấp trường: Hệ thống giám sát báo trộm cho xe máy
63 trang 205 0 0 -
NGHIÊN CỨU CHỌN TẠO CÁC GIỐNG LÚA CHẤT LƯỢNG CAO CHO VÙNG ĐỒNG BẰNG SÔNG CỬU LONG
9 trang 204 0 0