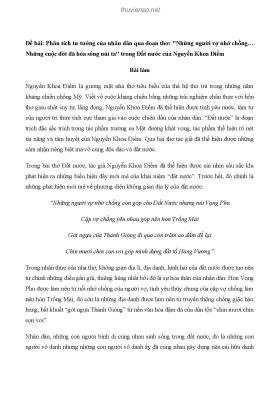
Báo cáo hóa học: Research Article On the Performance of Kernel Methods for Skin Color Segmentation
Số trang: 13
Loại file: pdf
Dung lượng: 2.25 MB
Lượt xem: 5
Lượt tải: 0
Xem trước 2 trang đầu tiên của tài liệu này:
Thông tin tài liệu:
Tuyển tập báo cáo các nghiên cứu khoa học quốc tế ngành hóa học dành cho các bạn yêu hóa học tham khảo đề tài: Research Article On the Performance of Kernel Methods for Skin Color Segmentation
Nội dung trích xuất từ tài liệu:
Báo cáo hóa học: " Research Article On the Performance of Kernel Methods for Skin Color Segmentation"Hindawi Publishing CorporationEURASIP Journal on Advances in Signal ProcessingVolume 2009, Article ID 856039, 13 pagesdoi:10.1155/2009/856039Research ArticleOn the Performance of Kernel Methods forSkin Color Segmentation ´ A. Guerrero-Curieses,1 J. L. Rojo-Alvarez,1 P. Conde-Pardo,2 I. Landesa-V´ zquez,2 a 1 and J. L. Alba-Castro2 ´ J. Ramos-Lopez, 1 Departamento de Teor´a de la Se˜ al y Comunicaciones, Universidad Rey Juan Carlos, 28943 Fuenlabrada, Spain ı n 2 Departamento de Teor´a de la Se˜ al y Comunicaciones, Universidad de Vigo, 36200 Vigo, Spain ı n Correspondence should be addressed to A. Guerrero-Curieses, alicia.guerrero@urjc.es Received 26 September 2008; Revised 23 March 2009; Accepted 7 May 2009 Recommended by C.-C. Kuo Human skin detection in color images is a key preprocessing stage in many image processing applications. Though kernel-based methods have been recently pointed out as advantageous for this setting, there is still few evidence on their actual superiority. Specifically, binary Support Vector Classifier (two-class SVM) and one-class Novelty Detection (SVND) have been only tested in some example images or in limited databases. We hypothesize that comparative performance evaluation on a representative application-oriented database will allow us to determine whether proposed kernel methods exhibit significant better performance than conventional skin segmentation methods. Two image databases were acquired for a webcam-based face recognition application, under controlled and uncontrolled lighting and background conditions. Three different chromaticity spaces (YCbCr, CIEL∗ a∗ b∗ , and normalized RGB) were used to compare kernel methods (two-class SVM, SVND) with conventional algorithms (Gaussian Mixture Models and Neural Networks). Our results show that two-class SVM outperforms conventional classifiers and also one-class SVM (SVND) detectors, specially for uncontrolled lighting conditions, with an acceptably low complexity. Copyright © 2009 A. Guerrero-Curieses et al. This is an open access article distributed under the Creative Commons Attribution License, which permits unrestricted use, distribution, and reproduction in any medium, provided the original work is properly cited.1. Introduction both the skin and the nonskin classes [12]. Even with an accurate estimation of the parameters in any density-basedSkin detection is often the first step in many image processing parametric models, the best detection rate in skin colorman-machine applications, such as face detection [1, 2], segmentation cannot be ensured. When a nonparametricgesture recognition [3], video surveillance [4], human modeling is adopted instead, a relatively high number ofvideo tracking [5], or adaptive video coding [6]. Although samples is required for an accurate representation of skin andpixelwise skin color alone is not sufficient for segmenting nonskin regions, like histograms [13] or Neural Networkshuman faces or hands, color segmentation for skin detection (NN) [12].has been proven to be an effective preprocessing step for Recently, the suitability of kernel methods has beenthe subsequent processing analysis. The segmentation task pointed out as an alternative approach for skin segmentationin most of the skin detection literature is achieved by in color spaces [14–17]. First, the Support Vector Machineusing simple thresholding [7], histogram analysis [8], single (SVM) was proposed for classifying pixels into skin orGaussian distribution models [9], or Gaussian Mixture nonskin samples, by stating the segmentation problem asModels (GMM) [1, 10, 11]. The main drawbacks of the a binary classification task [17], and later, some authorsdistribution-based parametric modeling techniques are, first, have proposed that the main interest in skin segmentationtheir strong dependence on the chosen color space and could be an adequate description of the domain thatlighting conditions, and second, the need for selection of supports the skin pixels in the space color, rather than devoting effort to model the more heterogeneous nonskinthe appropriate model for statistical characterization of2 EURASIP Journal on Advances in Signal Processingclass [14, 15]. According to this hypothesis, one-class kernel several color space transformations have been proposed andalgorithms, known in the kernel literature as Support Vector compared [7, 10, 17, 20], none of them can be considered asNovelty Detection (SVND) [18, 19], have been used for skin the optimal one. The selection of an adequate color space issegmentation. largely dependent on factors like the robustness to changing However, and to our best knowledge, few exhaustive per- illumination spectra, the selection of a suitable distributionformance comparison have been made ...
Nội dung trích xuất từ tài liệu:
Báo cáo hóa học: " Research Article On the Performance of Kernel Methods for Skin Color Segmentation"Hindawi Publishing CorporationEURASIP Journal on Advances in Signal ProcessingVolume 2009, Article ID 856039, 13 pagesdoi:10.1155/2009/856039Research ArticleOn the Performance of Kernel Methods forSkin Color Segmentation ´ A. Guerrero-Curieses,1 J. L. Rojo-Alvarez,1 P. Conde-Pardo,2 I. Landesa-V´ zquez,2 a 1 and J. L. Alba-Castro2 ´ J. Ramos-Lopez, 1 Departamento de Teor´a de la Se˜ al y Comunicaciones, Universidad Rey Juan Carlos, 28943 Fuenlabrada, Spain ı n 2 Departamento de Teor´a de la Se˜ al y Comunicaciones, Universidad de Vigo, 36200 Vigo, Spain ı n Correspondence should be addressed to A. Guerrero-Curieses, alicia.guerrero@urjc.es Received 26 September 2008; Revised 23 March 2009; Accepted 7 May 2009 Recommended by C.-C. Kuo Human skin detection in color images is a key preprocessing stage in many image processing applications. Though kernel-based methods have been recently pointed out as advantageous for this setting, there is still few evidence on their actual superiority. Specifically, binary Support Vector Classifier (two-class SVM) and one-class Novelty Detection (SVND) have been only tested in some example images or in limited databases. We hypothesize that comparative performance evaluation on a representative application-oriented database will allow us to determine whether proposed kernel methods exhibit significant better performance than conventional skin segmentation methods. Two image databases were acquired for a webcam-based face recognition application, under controlled and uncontrolled lighting and background conditions. Three different chromaticity spaces (YCbCr, CIEL∗ a∗ b∗ , and normalized RGB) were used to compare kernel methods (two-class SVM, SVND) with conventional algorithms (Gaussian Mixture Models and Neural Networks). Our results show that two-class SVM outperforms conventional classifiers and also one-class SVM (SVND) detectors, specially for uncontrolled lighting conditions, with an acceptably low complexity. Copyright © 2009 A. Guerrero-Curieses et al. This is an open access article distributed under the Creative Commons Attribution License, which permits unrestricted use, distribution, and reproduction in any medium, provided the original work is properly cited.1. Introduction both the skin and the nonskin classes [12]. Even with an accurate estimation of the parameters in any density-basedSkin detection is often the first step in many image processing parametric models, the best detection rate in skin colorman-machine applications, such as face detection [1, 2], segmentation cannot be ensured. When a nonparametricgesture recognition [3], video surveillance [4], human modeling is adopted instead, a relatively high number ofvideo tracking [5], or adaptive video coding [6]. Although samples is required for an accurate representation of skin andpixelwise skin color alone is not sufficient for segmenting nonskin regions, like histograms [13] or Neural Networkshuman faces or hands, color segmentation for skin detection (NN) [12].has been proven to be an effective preprocessing step for Recently, the suitability of kernel methods has beenthe subsequent processing analysis. The segmentation task pointed out as an alternative approach for skin segmentationin most of the skin detection literature is achieved by in color spaces [14–17]. First, the Support Vector Machineusing simple thresholding [7], histogram analysis [8], single (SVM) was proposed for classifying pixels into skin orGaussian distribution models [9], or Gaussian Mixture nonskin samples, by stating the segmentation problem asModels (GMM) [1, 10, 11]. The main drawbacks of the a binary classification task [17], and later, some authorsdistribution-based parametric modeling techniques are, first, have proposed that the main interest in skin segmentationtheir strong dependence on the chosen color space and could be an adequate description of the domain thatlighting conditions, and second, the need for selection of supports the skin pixels in the space color, rather than devoting effort to model the more heterogeneous nonskinthe appropriate model for statistical characterization of2 EURASIP Journal on Advances in Signal Processingclass [14, 15]. According to this hypothesis, one-class kernel several color space transformations have been proposed andalgorithms, known in the kernel literature as Support Vector compared [7, 10, 17, 20], none of them can be considered asNovelty Detection (SVND) [18, 19], have been used for skin the optimal one. The selection of an adequate color space issegmentation. largely dependent on factors like the robustness to changing However, and to our best knowledge, few exhaustive per- illumination spectra, the selection of a suitable distributionformance comparison have been made ...
Tìm kiếm theo từ khóa liên quan:
báo cáo khoa học báo cáo hóa học công trình nghiên cứu về hóa học tài liệu về hóa học cách trình bày báo cáoTài liệu liên quan:
-
HƯỚNG DẪN THỰC TẬP VÀ VIẾT BÁO CÁO THỰC TẬP TỐT NGHIỆP
18 trang 361 0 0 -
63 trang 334 0 0
-
13 trang 268 0 0
-
Báo cáo khoa học Bước đầu tìm hiểu văn hóa ẩm thực Trà Vinh
61 trang 255 0 0 -
Hướng dẫn thực tập tốt nghiệp dành cho sinh viên đại học Ngành quản trị kinh doanh
20 trang 248 0 0 -
Tóm tắt luận án tiến sỹ Một số vấn đề tối ưu hóa và nâng cao hiệu quả trong xử lý thông tin hình ảnh
28 trang 225 0 0 -
Đồ án: Nhà máy thủy điện Vĩnh Sơn - Bình Định
54 trang 223 0 0 -
23 trang 217 0 0
-
NGHIÊN CỨU CHỌN TẠO CÁC GIỐNG LÚA CHẤT LƯỢNG CAO CHO VÙNG ĐỒNG BẰNG SÔNG CỬU LONG
9 trang 216 0 0 -
Đề tài nghiên cứu khoa học và công nghệ cấp trường: Hệ thống giám sát báo trộm cho xe máy
63 trang 214 0 0