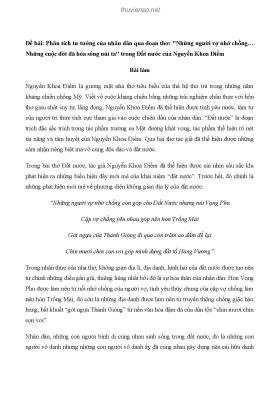
Báo cáo hóa học: Research Article Phoneme and Sentence-Level Ensembles for Speech Recognition
Số trang: 17
Loại file: pdf
Dung lượng: 1.16 MB
Lượt xem: 4
Lượt tải: 0
Xem trước 2 trang đầu tiên của tài liệu này:
Thông tin tài liệu:
Tuyển tập báo cáo các nghiên cứu khoa học quốc tế ngành hóa học dành cho các bạn yêu hóa học tham khảo đề tài: Research Article Phoneme and Sentence-Level Ensembles for Speech Recognition
Nội dung trích xuất từ tài liệu:
Báo cáo hóa học: " Research Article Phoneme and Sentence-Level Ensembles for Speech Recognition"Hindawi Publishing CorporationEURASIP Journal on Audio, Speech, and Music ProcessingVolume 2011, Article ID 426792, 17 pagesdoi:10.1155/2011/426792Research ArticlePhoneme and Sentence-Level Ensembles for Speech Recognition Christos Dimitrakakis1 and Samy Bengio2 1 FIAS, Ruth-Moufang-Strß 1, 60438 Frankfurt, Germany 2 Google, 1600 Amphitheatre Parkway, B1350-138, Mountain View, CA 94043, USA Correspondence should be addressed to Christos Dimitrakakis, christos.dimitrakakis@gmail.com Received 17 September 2010; Accepted 20 January 2011 Academic Editor: Elmar N¨ th o Copyright © 2011 C. Dimitrakakis and S. Bengio. This is an open access article distributed under the Creative Commons Attribution License, which permits unrestricted use, distribution, and reproduction in any medium, provided the original work is properly cited. We address the question of whether and how boosting and bagging can be used for speech recognition. In order to do this, we compare two different boosting schemes, one at the phoneme level and one at the utterance level, with a phoneme-level bagging scheme. We control for many parameters and other choices, such as the state inference scheme used. In an unbiased experiment, we clearly show that the gain of boosting methods compared to a single hidden Markov model is in all cases only marginal, while bagging significantly outperforms all other methods. We thus conclude that bagging methods, which have so far been overlooked in favour of boosting, should be examined more closely as a potentially useful ensemble learning technique for speech recognition.1. Introduction Section 2 introduces notation and provides some back- ground to speech recognition using hidden Markov models.This paper examines the application of ensemble methods to In addition, it discusses multistream methods for combininghidden Markov models (HMMs) for speech recognition. We multiple hidden Markov models to perform speech recogni-consider two methods: bagging and boosting. Both methods tion. Finally, it introduces the ensemble methods used in thefeature a fixed mixing distribution between the ensemble paper, bagging and boosting, in their basic form.components, which simplifies the inference, though it does Section 3 discusses related work and their relation to ournot completely trivialise it. contributions, while Section 4 gives details about the data This paper follows up on and consolidates previous and the experimental protocols followed.results [1–3] that focused on boosting. The main con- In the speech model considered, words are hiddentributions are the following. Firstly, we use an unbiased Markov models composed of concatenations of phoneticmodel testing methodology to perform the experimental hidden Markov models. In this setting it is possible to employcomparison between the various different approaches. A mixture models at any temporal level. Section 5 considerslarger number of experiments, with additional experiments mixtures at the phoneme model level, where data with aon triphones, shed some further light on previous results phonetic segmentation is available. We can then restrict[2, 3]. Secondly, the results indicate that, in an unbiased ourselves to a sequence classification problem in order tocomparison, at least for the dataset and features considered, train a mixture model. Application of methods such asbagging approaches enjoy a significant advantage to boosting bagging and boosting to the phoneme classification taskapproaches. More specifically, bagging consistently exhibited ...
Nội dung trích xuất từ tài liệu:
Báo cáo hóa học: " Research Article Phoneme and Sentence-Level Ensembles for Speech Recognition"Hindawi Publishing CorporationEURASIP Journal on Audio, Speech, and Music ProcessingVolume 2011, Article ID 426792, 17 pagesdoi:10.1155/2011/426792Research ArticlePhoneme and Sentence-Level Ensembles for Speech Recognition Christos Dimitrakakis1 and Samy Bengio2 1 FIAS, Ruth-Moufang-Strß 1, 60438 Frankfurt, Germany 2 Google, 1600 Amphitheatre Parkway, B1350-138, Mountain View, CA 94043, USA Correspondence should be addressed to Christos Dimitrakakis, christos.dimitrakakis@gmail.com Received 17 September 2010; Accepted 20 January 2011 Academic Editor: Elmar N¨ th o Copyright © 2011 C. Dimitrakakis and S. Bengio. This is an open access article distributed under the Creative Commons Attribution License, which permits unrestricted use, distribution, and reproduction in any medium, provided the original work is properly cited. We address the question of whether and how boosting and bagging can be used for speech recognition. In order to do this, we compare two different boosting schemes, one at the phoneme level and one at the utterance level, with a phoneme-level bagging scheme. We control for many parameters and other choices, such as the state inference scheme used. In an unbiased experiment, we clearly show that the gain of boosting methods compared to a single hidden Markov model is in all cases only marginal, while bagging significantly outperforms all other methods. We thus conclude that bagging methods, which have so far been overlooked in favour of boosting, should be examined more closely as a potentially useful ensemble learning technique for speech recognition.1. Introduction Section 2 introduces notation and provides some back- ground to speech recognition using hidden Markov models.This paper examines the application of ensemble methods to In addition, it discusses multistream methods for combininghidden Markov models (HMMs) for speech recognition. We multiple hidden Markov models to perform speech recogni-consider two methods: bagging and boosting. Both methods tion. Finally, it introduces the ensemble methods used in thefeature a fixed mixing distribution between the ensemble paper, bagging and boosting, in their basic form.components, which simplifies the inference, though it does Section 3 discusses related work and their relation to ournot completely trivialise it. contributions, while Section 4 gives details about the data This paper follows up on and consolidates previous and the experimental protocols followed.results [1–3] that focused on boosting. The main con- In the speech model considered, words are hiddentributions are the following. Firstly, we use an unbiased Markov models composed of concatenations of phoneticmodel testing methodology to perform the experimental hidden Markov models. In this setting it is possible to employcomparison between the various different approaches. A mixture models at any temporal level. Section 5 considerslarger number of experiments, with additional experiments mixtures at the phoneme model level, where data with aon triphones, shed some further light on previous results phonetic segmentation is available. We can then restrict[2, 3]. Secondly, the results indicate that, in an unbiased ourselves to a sequence classification problem in order tocomparison, at least for the dataset and features considered, train a mixture model. Application of methods such asbagging approaches enjoy a significant advantage to boosting bagging and boosting to the phoneme classification taskapproaches. More specifically, bagging consistently exhibited ...
Tìm kiếm theo từ khóa liên quan:
báo cáo khoa học báo cáo hóa học công trình nghiên cứu về hóa học tài liệu về hóa học cách trình bày báo cáoTài liệu liên quan:
-
HƯỚNG DẪN THỰC TẬP VÀ VIẾT BÁO CÁO THỰC TẬP TỐT NGHIỆP
18 trang 362 0 0 -
63 trang 340 0 0
-
13 trang 268 0 0
-
Báo cáo khoa học Bước đầu tìm hiểu văn hóa ẩm thực Trà Vinh
61 trang 255 0 0 -
Hướng dẫn thực tập tốt nghiệp dành cho sinh viên đại học Ngành quản trị kinh doanh
20 trang 253 0 0 -
Tóm tắt luận án tiến sỹ Một số vấn đề tối ưu hóa và nâng cao hiệu quả trong xử lý thông tin hình ảnh
28 trang 227 0 0 -
Đồ án: Nhà máy thủy điện Vĩnh Sơn - Bình Định
54 trang 223 0 0 -
23 trang 219 0 0
-
NGHIÊN CỨU CHỌN TẠO CÁC GIỐNG LÚA CHẤT LƯỢNG CAO CHO VÙNG ĐỒNG BẰNG SÔNG CỬU LONG
9 trang 218 0 0 -
Đề tài nghiên cứu khoa học và công nghệ cấp trường: Hệ thống giám sát báo trộm cho xe máy
63 trang 214 0 0