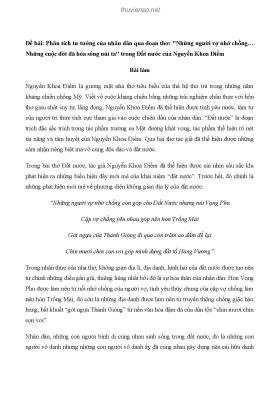
Exploring the behavior patterns of students accessing online learning material using cluster analysis
Số trang: 11
Loại file: pdf
Dung lượng: 1.27 MB
Lượt xem: 15
Lượt tải: 0
Xem trước 2 trang đầu tiên của tài liệu này:
Thông tin tài liệu:
Understanding students’ behavior in online courses may provide teachers with useful information to improve their educational design and provide insights for content and instructional designers to develop personalized learning support. This research uses cluster analysis to explore learners’ interaction with online learning materials behavior in an online course at Hung Vuong University and identified three clusters (Less-engaged students, Moderately-engaged students, and Highly-engaged students) which evince different behavior patterns with regards to the time spent interacting with various resources. Based on the findings, several suggestions are also proposed for future research.
Nội dung trích xuất từ tài liệu:
Exploring the behavior patterns of students accessing online learning material using cluster analysis TẠP TẠP CHÍ KHOA HỌC VÀCHÍ CÔNGKHOA NGHỆHỌC VÀ CÔNG NGHỆ JOURNAL OF SCIENCE AND TECHNOLOGY Pham Duc Tho TRƯỜNG ĐẠI HỌC HÙNG VƯƠNG HUNG VUONG UNIVERSITY Tập 28, Số 3 (2022): 58-68 Vol. 28, No. 3 (2022): 58-68 Email: tapchikhoahoc@hvu.edu.vn Website: www.hvu.edu.vn EXPLORING THE BEHAVIOR PATTERNS OF STUDENTS ACCESSING ONLINE LEARNING MATERIAL USING CLUSTER ANALYSIS Pham Duc Tho1* 1 Faculyty of Engineering and Technology, Hung Vuong University, Phu Tho Received: 08 Ferbuary 2022; Revised: 10 March 2022; Accepted: 10 May 2022AbstractU nderstanding students’ behavior in online courses may provide teachers with useful information to improve their educational design and provide insights for content and instructional designers to developpersonalized learning support. This research uses cluster analysis to explore learners’ interaction with onlinelearning materials behavior in an online course at Hung Vuong University and identified three clusters (Less-engaged students, Moderately-engaged students, and Highly-engaged students) which evince different behaviorpatterns with regards to the time spent interacting with various resources. Based on the findings, severalsuggestions are also proposed for future research.Keywords: Students behavior, online courses, cluster analysis, behavior patterns.1. Introduction resources for self-reflection [4, 5]. Students It is well recognized that the interaction may demonstrate different levels ofwith online learning materials is one of the engagement and patterns of behavior whenmost commonly performed online learning interacting with online learning materialsactivities [1]. Teachers and students typically for different purposes and based on differentpublish and create different kinds of online preferences [1, 6]; these levels of engagementresources for learning, and such materials and patterns of behavior may, in turn, affectgive different learning advantages. For their learning performance [6, 7].example, lecture slides give an outline of Consequently, understanding howteaching contents for students and facilitate students interact with different types ofstudents’ note-taking [2], students may learning materials and how their behavior inreview challenging concepts and prepare interacting with these materials affects theirfor examinations through video lectures learning performance may provide teachers[3], while peers’ assignments and messages with useful information to improve theirposted in discussion forums are essential educational design and provide insights for58 *Email: thopham@hvu.edu.vnTẠP CHÍ KHOA HỌC VÀ CÔNG NGHỆ Tập 28, Số 3 (2022): 58-68content and instructional designers to develop how different interaction behaviors affectpersonalized learning support. However, learning performance. Heffner and Cohenonly using statistical methods is not enough [16] examined the relationships betweento explore students’ interaction with online the behaviors of viewing online courselearning material behavior. Cluster analysis materials (e.g., number of viewing syllabus,(e.g., the K-means method) can be used to number of viewing course information, andinvestigate the behavior cluster patterns of number of viewing instructor information),a group regarding various indicators (such the individual difference (gender), and finalas the frequency of a particular discussion course grade. They found that the numberbehavior) [8]. Thus, the use of clustering of times course materials were accessedtechniques on these behavior sets enables the had a positive relationship with the finalpotential cluster patterns of learners’ different course grade and that female students m ...
Nội dung trích xuất từ tài liệu:
Exploring the behavior patterns of students accessing online learning material using cluster analysis TẠP TẠP CHÍ KHOA HỌC VÀCHÍ CÔNGKHOA NGHỆHỌC VÀ CÔNG NGHỆ JOURNAL OF SCIENCE AND TECHNOLOGY Pham Duc Tho TRƯỜNG ĐẠI HỌC HÙNG VƯƠNG HUNG VUONG UNIVERSITY Tập 28, Số 3 (2022): 58-68 Vol. 28, No. 3 (2022): 58-68 Email: tapchikhoahoc@hvu.edu.vn Website: www.hvu.edu.vn EXPLORING THE BEHAVIOR PATTERNS OF STUDENTS ACCESSING ONLINE LEARNING MATERIAL USING CLUSTER ANALYSIS Pham Duc Tho1* 1 Faculyty of Engineering and Technology, Hung Vuong University, Phu Tho Received: 08 Ferbuary 2022; Revised: 10 March 2022; Accepted: 10 May 2022AbstractU nderstanding students’ behavior in online courses may provide teachers with useful information to improve their educational design and provide insights for content and instructional designers to developpersonalized learning support. This research uses cluster analysis to explore learners’ interaction with onlinelearning materials behavior in an online course at Hung Vuong University and identified three clusters (Less-engaged students, Moderately-engaged students, and Highly-engaged students) which evince different behaviorpatterns with regards to the time spent interacting with various resources. Based on the findings, severalsuggestions are also proposed for future research.Keywords: Students behavior, online courses, cluster analysis, behavior patterns.1. Introduction resources for self-reflection [4, 5]. Students It is well recognized that the interaction may demonstrate different levels ofwith online learning materials is one of the engagement and patterns of behavior whenmost commonly performed online learning interacting with online learning materialsactivities [1]. Teachers and students typically for different purposes and based on differentpublish and create different kinds of online preferences [1, 6]; these levels of engagementresources for learning, and such materials and patterns of behavior may, in turn, affectgive different learning advantages. For their learning performance [6, 7].example, lecture slides give an outline of Consequently, understanding howteaching contents for students and facilitate students interact with different types ofstudents’ note-taking [2], students may learning materials and how their behavior inreview challenging concepts and prepare interacting with these materials affects theirfor examinations through video lectures learning performance may provide teachers[3], while peers’ assignments and messages with useful information to improve theirposted in discussion forums are essential educational design and provide insights for58 *Email: thopham@hvu.edu.vnTẠP CHÍ KHOA HỌC VÀ CÔNG NGHỆ Tập 28, Số 3 (2022): 58-68content and instructional designers to develop how different interaction behaviors affectpersonalized learning support. However, learning performance. Heffner and Cohenonly using statistical methods is not enough [16] examined the relationships betweento explore students’ interaction with online the behaviors of viewing online courselearning material behavior. Cluster analysis materials (e.g., number of viewing syllabus,(e.g., the K-means method) can be used to number of viewing course information, andinvestigate the behavior cluster patterns of number of viewing instructor information),a group regarding various indicators (such the individual difference (gender), and finalas the frequency of a particular discussion course grade. They found that the numberbehavior) [8]. Thus, the use of clustering of times course materials were accessedtechniques on these behavior sets enables the had a positive relationship with the finalpotential cluster patterns of learners’ different course grade and that female students m ...
Tìm kiếm theo từ khóa liên quan:
Online learning materials Online learning method Online courses for women Cluster analysis method Tài nguyên học liệu online Khóa học trực tuyến Tạp chí Khoa học và Công nghệTài liệu liên quan:
-
15 trang 224 0 0
-
9 trang 161 0 0
-
Phân tích và so sánh các loại pin sử dụng cho ô tô điện
6 trang 111 0 0 -
10 trang 90 0 0
-
Hội nhập quốc tế trong lĩnh vực pháp luật sở hữu trí tuệ của Việt Nam
4 trang 85 0 0 -
Ảnh hưởng các tham số trong bảng sam điều kiện đối với phương pháp điều khiển sử dụng đại số gia tử
9 trang 69 0 0 -
5 trang 69 0 0
-
15 trang 55 0 0
-
Đánh giá việc sử dụng xi măng thay thế bột khoáng nhằm cải thiện tính năng của bê tông nhựa nóng
5 trang 54 0 0 -
Mô hình quá trình kết tụ hạt dưới ảnh hưởng của sóng siêu âm trong hệ thống lọc bụi ly tâm
4 trang 50 0 0