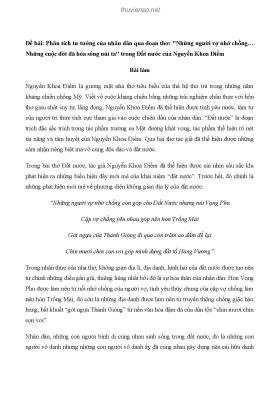
Hiệu quả của các phương pháp xử lý dữ liệu mất cân bằng trong chấm điểm tín dụng: Trường hợp tại các Ngân hàng thương mại Việt Nam
Số trang: 15
Loại file: pdf
Dung lượng: 284.83 KB
Lượt xem: 12
Lượt tải: 0
Xem trước 2 trang đầu tiên của tài liệu này:
Thông tin tài liệu:
Bài viết này nghiên cứu hiệu quả của phương pháp xử lý dữ liệu mất cân bằng trong bài toán phân loại khách hàng tại các ngân hàng thương mại. Đây là một vấn đề phổ biến trong vấn đề phân loại khách hàng, trong đó các quan sát của một lớp nhiều hơn lớp còn lại trong dữ liệu. Mời các bạn cùng tham khảo!
Nội dung trích xuất từ tài liệu:
Hiệu quả của các phương pháp xử lý dữ liệu mất cân bằng trong chấm điểm tín dụng: Trường hợp tại các Ngân hàng thương mại Việt Nam INTERNATIONAL CONFERENCE FOR YOUNG RESEARCHERS IN ECONOMICS & BUSINESS 2020 ICYREB 2020 THE EFFECTIVENESS OF METHODS IN DEALING WITH IMBALANCED DATA IN CREDIT SCORING: THE CASE OF VIETNAM COMMERCIAL BANKS HIỆU QUẢ CỦA CÁC PHƯƠNG PHÁP XỬ LÝ DỮ LIỆU MẤT CÂN BẰNG TRONG CHẤM ĐIỂM TÍN DỤNG: TRƯỜNG HỢP TẠI CÁC NGÂN HÀNG THƯƠNG MẠI VIỆT NAM Nguyen Thi Lien, MS ; Nguyen Thi Thu Trang, MS; Nguyen Thi Dung National Economics University lientkt@neu.edu.vn Abstract This article investigates the effectiveness of imbalanced data processing methods in the problem of customer classification at commercial banks. This is a common issue in a customer classification attempt, where observations of one class outnumber the remaining class. We apply the methods widely used in the world including undersampling, oversampling, bothsamling tech- niques, and SMOTE (Synthetic Minority Oversampling Technique) to deal with imbalances. The logit model is applied to datasets that have been processed by these methods to classify customers. Using 7501 transaction data from individual customers, the classification results using data processed with these techniques all improve significantly compared to using untreated data. Be- sides, the results also show that the most efficient method is SMOTE technique combined with the logit model using variables transformed by Weight of Evidence (WOE). Keywords: Bothsampling, credit scoring, oversampling, SMOTE, undersampling, WOE. Tóm tắt Bài báo này nghiên cứu hiệu quả của phương pháp xử lý dữ liệu mất cân bằng trong bài toán phân loại khách hàng tại các ngân hàng thương mại. Đây là một vấn đề phổ biến trong vấn đề phân loại khách hàng, trong đó các quan sát của một lớp nhiều hơn lớp còn lại trong dữ liệu. Chúng tôi áp dụng các phương pháp được sử dụng rộng rãi trên thế giới bao gồm kỹ thuật lấy mẫu dưới (Undersampling), lấy mẫu quá mức (Oversampling), kỹ thuật lấy mẫu cả hai (booth- sampling) và SMOTE (Synthetic Minority Oversampling Technique) để giải quyết vấn đề mất cân bằng. Mô hình logit được áp dụng cho các tập dữ liệu đã được xử lý mất cân bằng để phân loại khách hàng. Sử dụng 7501 dữ liệu giao dịch từ các khách hàng cá nhân, kết quả phân loại sử dụng dữ liệu đã được xử lý mất cân bằng đều cải thiện đáng kể so với sử dụng dữ liệu không được xử lý. Bên cạnh đó, kết quả cũng cho thấy phương pháp hiệu quả nhất là kỹ thuật SMOTE kết hợp với mô hình logit sử dụng các biến đầu vào được chuyển đổi sang trọng số bằng chứng (Weight of Evidence - WOE). Từ khóa: Chấm điểm tín dụng, lấy mẫu cả hai, lấy mẫu quá mức, lấy mẫu dưới, SMOTE, WOE 1273 INTERNATIONAL CONFERENCE FOR YOUNG RESEARCHERS IN ECONOMICS & BUSINESS 2020 ICYREB 2020 1. Introduction In the case of classification algorithms, we aim to predict the false observations with the highest accuracy. However, the accuracy of these algorithms can be influenced by the imbalanced data (López, 2013), where observations of one class outnumber the other class(es) at least 5:1 for the binary case (He & Garcia, 2009). In order to improve the prediction accuracy and lower the computation expense, a previous identification and quantification of the most relevant input variables of the model is always highly advised. Imbalanced data also appears in some other fields, such as fault detection (Gong & Qiao, 2012; Silva et al., 2006; Wei et al., 2013), toxin de- tection (Harley et al., 2020), medical diagnosis (Ertekin et al., 2007) and customer churn predic- tion (Bing Zhu et al., 2017). In medical area, consider the group of cancer patients as the positive class and remaining persons are in the negative class, the difference about quantity between these two groups in datasets is large and unequal in the number of observations between the groups. Other examples of rare events include software defects (Rodriguez et al., 2014), cancer gene ex- pressions (Wu et al., 2012), credit card transactions fraudulent (Kundu et al., 2009), fraud detec- tion in telecommunication (Augustin, 2012), and natural disaster events (Kim et al., 2016). The most prevalent class is called the majority class, while the rarest class is called the minority class (Huang et al., 2016). Several techniques to process imbalanced data are developed and applied on the aspect of data while others concentrate on the algorithmic level. To address the imbalanced problem, four categories in the applications include the preprocessing strategies, cost-sensitive learning meth- ods, adaptation of machine learnin ...
Nội dung trích xuất từ tài liệu:
Hiệu quả của các phương pháp xử lý dữ liệu mất cân bằng trong chấm điểm tín dụng: Trường hợp tại các Ngân hàng thương mại Việt Nam INTERNATIONAL CONFERENCE FOR YOUNG RESEARCHERS IN ECONOMICS & BUSINESS 2020 ICYREB 2020 THE EFFECTIVENESS OF METHODS IN DEALING WITH IMBALANCED DATA IN CREDIT SCORING: THE CASE OF VIETNAM COMMERCIAL BANKS HIỆU QUẢ CỦA CÁC PHƯƠNG PHÁP XỬ LÝ DỮ LIỆU MẤT CÂN BẰNG TRONG CHẤM ĐIỂM TÍN DỤNG: TRƯỜNG HỢP TẠI CÁC NGÂN HÀNG THƯƠNG MẠI VIỆT NAM Nguyen Thi Lien, MS ; Nguyen Thi Thu Trang, MS; Nguyen Thi Dung National Economics University lientkt@neu.edu.vn Abstract This article investigates the effectiveness of imbalanced data processing methods in the problem of customer classification at commercial banks. This is a common issue in a customer classification attempt, where observations of one class outnumber the remaining class. We apply the methods widely used in the world including undersampling, oversampling, bothsamling tech- niques, and SMOTE (Synthetic Minority Oversampling Technique) to deal with imbalances. The logit model is applied to datasets that have been processed by these methods to classify customers. Using 7501 transaction data from individual customers, the classification results using data processed with these techniques all improve significantly compared to using untreated data. Be- sides, the results also show that the most efficient method is SMOTE technique combined with the logit model using variables transformed by Weight of Evidence (WOE). Keywords: Bothsampling, credit scoring, oversampling, SMOTE, undersampling, WOE. Tóm tắt Bài báo này nghiên cứu hiệu quả của phương pháp xử lý dữ liệu mất cân bằng trong bài toán phân loại khách hàng tại các ngân hàng thương mại. Đây là một vấn đề phổ biến trong vấn đề phân loại khách hàng, trong đó các quan sát của một lớp nhiều hơn lớp còn lại trong dữ liệu. Chúng tôi áp dụng các phương pháp được sử dụng rộng rãi trên thế giới bao gồm kỹ thuật lấy mẫu dưới (Undersampling), lấy mẫu quá mức (Oversampling), kỹ thuật lấy mẫu cả hai (booth- sampling) và SMOTE (Synthetic Minority Oversampling Technique) để giải quyết vấn đề mất cân bằng. Mô hình logit được áp dụng cho các tập dữ liệu đã được xử lý mất cân bằng để phân loại khách hàng. Sử dụng 7501 dữ liệu giao dịch từ các khách hàng cá nhân, kết quả phân loại sử dụng dữ liệu đã được xử lý mất cân bằng đều cải thiện đáng kể so với sử dụng dữ liệu không được xử lý. Bên cạnh đó, kết quả cũng cho thấy phương pháp hiệu quả nhất là kỹ thuật SMOTE kết hợp với mô hình logit sử dụng các biến đầu vào được chuyển đổi sang trọng số bằng chứng (Weight of Evidence - WOE). Từ khóa: Chấm điểm tín dụng, lấy mẫu cả hai, lấy mẫu quá mức, lấy mẫu dưới, SMOTE, WOE 1273 INTERNATIONAL CONFERENCE FOR YOUNG RESEARCHERS IN ECONOMICS & BUSINESS 2020 ICYREB 2020 1. Introduction In the case of classification algorithms, we aim to predict the false observations with the highest accuracy. However, the accuracy of these algorithms can be influenced by the imbalanced data (López, 2013), where observations of one class outnumber the other class(es) at least 5:1 for the binary case (He & Garcia, 2009). In order to improve the prediction accuracy and lower the computation expense, a previous identification and quantification of the most relevant input variables of the model is always highly advised. Imbalanced data also appears in some other fields, such as fault detection (Gong & Qiao, 2012; Silva et al., 2006; Wei et al., 2013), toxin de- tection (Harley et al., 2020), medical diagnosis (Ertekin et al., 2007) and customer churn predic- tion (Bing Zhu et al., 2017). In medical area, consider the group of cancer patients as the positive class and remaining persons are in the negative class, the difference about quantity between these two groups in datasets is large and unequal in the number of observations between the groups. Other examples of rare events include software defects (Rodriguez et al., 2014), cancer gene ex- pressions (Wu et al., 2012), credit card transactions fraudulent (Kundu et al., 2009), fraud detec- tion in telecommunication (Augustin, 2012), and natural disaster events (Kim et al., 2016). The most prevalent class is called the majority class, while the rarest class is called the minority class (Huang et al., 2016). Several techniques to process imbalanced data are developed and applied on the aspect of data while others concentrate on the algorithmic level. To address the imbalanced problem, four categories in the applications include the preprocessing strategies, cost-sensitive learning meth- ods, adaptation of machine learnin ...
Tìm kiếm theo từ khóa liên quan:
Xử lý dữ liệu mất cân bằng Chấm điểm tín dụng Quản trị rủi ro tín dụng Ngân hàng thương mại Việt Nam Kỹ thuật lấy mẫu dướiGợi ý tài liệu liên quan:
-
Luận án Tiến sĩ Tài chính - Ngân hàng: Phát triển tín dụng xanh tại ngân hàng thương mại Việt Nam
267 trang 385 1 0 -
Lý thuyết, bài tập và bài giải Nghiệp vụ ngân hàng thương mại: Phần 2 - PGS.TS. Nguyễn Minh Kiều
299 trang 147 4 0 -
Tăng trưởng cho vay và sự an toàn của các ngân hàng thương mại Việt Nam
9 trang 123 0 0 -
Quản trị rủi ro tại các ngân hàng thương mại Việt Nam và những vấn đề đặt ra
5 trang 115 0 0 -
Hoạt động của các ngân hàng thương mại Việt Nam: Những vấn đề cần quan tâm hiện nay
6 trang 113 0 0 -
34 trang 101 0 0
-
19 trang 100 0 0
-
15 trang 92 0 0
-
72 trang 91 0 0
-
108 trang 81 0 0