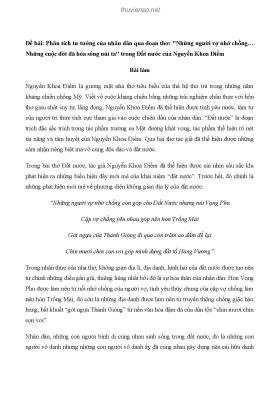
Networking Theory and Fundamentals - Lecture 3
Số trang: 36
Loại file: pdf
Dung lượng: 343.54 KB
Lượt xem: 18
Lượt tải: 0
Xem trước 4 trang đầu tiên của tài liệu này:
Thông tin tài liệu:
Tham khảo tài liệu 'networking theory and fundamentals - lecture 3', công nghệ thông tin, quản trị mạng phục vụ nhu cầu học tập, nghiên cứu và làm việc hiệu quả
Nội dung trích xuất từ tài liệu:
Networking Theory and Fundamentals - Lecture 3 TCOM 501: Networking Theory & Fundamentals Lecture 3 January 29, 2003 Prof. Yannis A. Korilis 1 Topics 3-2 Markov Chains Discrete-Time Markov Chains Calculating Stationary Distribution Global Balance Equations Detailed Balance Equations Birth-Death Process Generalized Markov Chains Continuous-Time Markov Chains Markov Chain 3-3 Stochastic process that takes values in a countable set Example: {0,1,2,…,m}, or {0,1,2,…} Elements represent possible “states” Chain “jumps” from state to state Memoryless (Markov) Property: Given the present state, future jumps of the chain are independent of past history Markov Chains: discrete- or continuous- time Discrete-Time Markov Chain 3-4 Discrete-time stochastic process {Xn: n = 0,1,2,…} Takes values in {0,1,2,…} Memoryless property: P{ X n +1 = j | X n = i, X n −1 = in −1 , ..., X 0 = i0 } = P{ X n +1 = j | X n = i} Pij = P{ X n +1 = j | X n = i} Transition probabilities Pij ∞ ∑P Pij ≥ 0, =1 ij j =0 Transition probability matrix P=[Pij] Chapman-Kolmogorov Equations 3-5 n step transition probabilities Pijn = P{ X n + m = j | X m = i}, n, m ≥ 0, i, j ≥ 0 Chapman-Kolmogorov equations ∞ = ∑ Pikn Pkm , n+m n, m ≥ 0, i, j ≥ 0 Pij j k =0 Pijn is element (i, j) in matrix Pn Recursive computation of state probabilities State Probabilities – Stationary Distribution 3-6 State probabilities (time-dependent) π nj = P{ X n = j}, π n = ( π 0 , π1 ,...) n n ∞ ∞ P{ X n = j} = ∑ P{ X n −1 = i}P{ X n = j | X n −1 = i} ⇒ π = ∑ π in −1Pij n j i =0 i =0 In matrix form: π n = π n −1P = π n −2 P 2 = ... = π 0 P n If time-dependent distribution converges to a limit π = lim π n n →∞ π is called the stationary distribution π = πP Existence depends on the structure of Markov chain Classification of Markov Chains 3-7 Irreducible: Aperiodic: States i and j communicate: State i is periodic: ∃n, m : Pijn > 0, Pji > 0 ∃ d > 1: Piin > 0 ⇒ n = α d m Irreducible Markov chain: all Aperiodic Markov chain: none states communicate of the states is periodic 1 2 1 2 0 0 3 4 3 4 Limit Theorems 3-8 Theorem 1: Irreducible aperiodic Markov chain For every state j, the following limit π j = lim P{ X n = j | X 0 = i}, i = 0,1, 2, ... n →∞ exists and is independent of initial state i Nj(k): number of visits to state j up to time k N j (k ) P π j = lim X0 = i = 1 k k →∞ πj: frequency the process visits state j Existence of Stationary Distribution 3-9 Theorem 2: Irreducible aperiodic Markov chain. There are two possibilities for scalars: π j = lim P{ X n = j | X 0 = i} = lim Pijn n →∞ n →∞ πj = 0, for all states j No stationary distribution 1. πj > 0, for all states j π is the unique stationary 2. distribution Remark: If the number of states is finite, case 2 is the only possibility Ergodic Markov Chains 3-10 Markov chain with a stationary distribution π j > 0, j = 0,1, 2, ... States are positive recurrent: The process returns to state j “infinitely often” A positive recurrent and aperiodic Markov chain is called ergodic Ergodic chains have a unique stationary distribution π j = lim Pijn n →∞ Ergodicity ⇒ Time Averages = Stochastic Averages Calculation of Stationary Distribution 3-11 A. Finite number of states B. Infinite number of states Solve explicitly the system of Cannot apply previous methods equations to problem of infinite dimension m Guess a solution to recurrence: π j = ∑ πi Pij , j = 0,1, ..., m ∞ π j = ∑ πi Pij , i =0 j = 0,1, ..., m ∑π =1 ...
Nội dung trích xuất từ tài liệu:
Networking Theory and Fundamentals - Lecture 3 TCOM 501: Networking Theory & Fundamentals Lecture 3 January 29, 2003 Prof. Yannis A. Korilis 1 Topics 3-2 Markov Chains Discrete-Time Markov Chains Calculating Stationary Distribution Global Balance Equations Detailed Balance Equations Birth-Death Process Generalized Markov Chains Continuous-Time Markov Chains Markov Chain 3-3 Stochastic process that takes values in a countable set Example: {0,1,2,…,m}, or {0,1,2,…} Elements represent possible “states” Chain “jumps” from state to state Memoryless (Markov) Property: Given the present state, future jumps of the chain are independent of past history Markov Chains: discrete- or continuous- time Discrete-Time Markov Chain 3-4 Discrete-time stochastic process {Xn: n = 0,1,2,…} Takes values in {0,1,2,…} Memoryless property: P{ X n +1 = j | X n = i, X n −1 = in −1 , ..., X 0 = i0 } = P{ X n +1 = j | X n = i} Pij = P{ X n +1 = j | X n = i} Transition probabilities Pij ∞ ∑P Pij ≥ 0, =1 ij j =0 Transition probability matrix P=[Pij] Chapman-Kolmogorov Equations 3-5 n step transition probabilities Pijn = P{ X n + m = j | X m = i}, n, m ≥ 0, i, j ≥ 0 Chapman-Kolmogorov equations ∞ = ∑ Pikn Pkm , n+m n, m ≥ 0, i, j ≥ 0 Pij j k =0 Pijn is element (i, j) in matrix Pn Recursive computation of state probabilities State Probabilities – Stationary Distribution 3-6 State probabilities (time-dependent) π nj = P{ X n = j}, π n = ( π 0 , π1 ,...) n n ∞ ∞ P{ X n = j} = ∑ P{ X n −1 = i}P{ X n = j | X n −1 = i} ⇒ π = ∑ π in −1Pij n j i =0 i =0 In matrix form: π n = π n −1P = π n −2 P 2 = ... = π 0 P n If time-dependent distribution converges to a limit π = lim π n n →∞ π is called the stationary distribution π = πP Existence depends on the structure of Markov chain Classification of Markov Chains 3-7 Irreducible: Aperiodic: States i and j communicate: State i is periodic: ∃n, m : Pijn > 0, Pji > 0 ∃ d > 1: Piin > 0 ⇒ n = α d m Irreducible Markov chain: all Aperiodic Markov chain: none states communicate of the states is periodic 1 2 1 2 0 0 3 4 3 4 Limit Theorems 3-8 Theorem 1: Irreducible aperiodic Markov chain For every state j, the following limit π j = lim P{ X n = j | X 0 = i}, i = 0,1, 2, ... n →∞ exists and is independent of initial state i Nj(k): number of visits to state j up to time k N j (k ) P π j = lim X0 = i = 1 k k →∞ πj: frequency the process visits state j Existence of Stationary Distribution 3-9 Theorem 2: Irreducible aperiodic Markov chain. There are two possibilities for scalars: π j = lim P{ X n = j | X 0 = i} = lim Pijn n →∞ n →∞ πj = 0, for all states j No stationary distribution 1. πj > 0, for all states j π is the unique stationary 2. distribution Remark: If the number of states is finite, case 2 is the only possibility Ergodic Markov Chains 3-10 Markov chain with a stationary distribution π j > 0, j = 0,1, 2, ... States are positive recurrent: The process returns to state j “infinitely often” A positive recurrent and aperiodic Markov chain is called ergodic Ergodic chains have a unique stationary distribution π j = lim Pijn n →∞ Ergodicity ⇒ Time Averages = Stochastic Averages Calculation of Stationary Distribution 3-11 A. Finite number of states B. Infinite number of states Solve explicitly the system of Cannot apply previous methods equations to problem of infinite dimension m Guess a solution to recurrence: π j = ∑ πi Pij , j = 0,1, ..., m ∞ π j = ∑ πi Pij , i =0 j = 0,1, ..., m ∑π =1 ...
Tài liệu liên quan:
-
Giáo án Tin học lớp 9 (Trọn bộ cả năm)
149 trang 280 0 0 -
Giáo trình Hệ thống mạng máy tính CCNA (Tập 4): Phần 2
102 trang 261 0 0 -
Ngân hàng câu hỏi trắc nghiệm môn mạng máy tính
99 trang 260 1 0 -
Bài giảng: Lịch sử phát triển hệ thống mạng
118 trang 260 0 0 -
73 trang 249 0 0
-
47 trang 242 3 0
-
Đề cương chi tiết học phần Thiết kế và cài đặt mạng
3 trang 241 0 0 -
80 trang 230 0 0
-
Giáo trình Hệ thống mạng máy tính CCNA (Tập 4): Phần 1
122 trang 219 0 0 -
122 trang 217 0 0