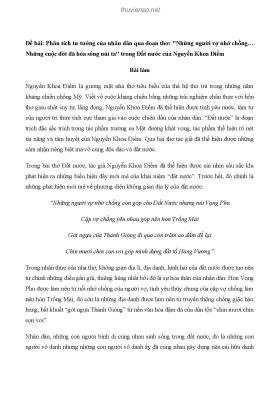
Ứng dụng chuỗi Markov để dự đoán xu hướng của thị trường
Số trang: 7
Loại file: pdf
Dung lượng: 455.06 KB
Lượt xem: 16
Lượt tải: 0
Xem trước 2 trang đầu tiên của tài liệu này:
Thông tin tài liệu:
Bài viết "Ứng dụng chuỗi Markov để dự đoán xu hướng của thị trường" trình bày các khái niệm và tính chất của chuỗi Markov và đề cập đến một số ứng dụng của chúng trong hoạt động kinh doanh, kinh tế thương mại như dự đoán các xu hướng phát triển của thị trường. Mời các bạn cùng tham khảo chi tiết nội dung bài viết!
Nội dung trích xuất từ tài liệu:
Ứng dụng chuỗi Markov để dự đoán xu hướng của thị trường ỨNG DỤNG CHUỖI MARKOV ĐỂ DỰ ĐOÁN XU HƯỚNG CỦA THỊ TRƯỜNG Nguyễn Thị Hương Trường Đại học Hà Nội Tóm tắt - Khái niệm chuỗi Markov lần đầu tiên được đề cập bởi nhà Toán học người NgaA.Markov (1856 - 1922) vào năm 1906. Ngày nay chuỗi Markov được ứng dụng rộng rãi trongnhiều lĩnh vực khoa học khác nhau, như Thống kê, lý thuyết hàng chờ, logistics,…Chuỗi Markovthường được dùng để mô phỏng hệ thống phục vụ trong mối liên hệ với các tiến trình Poison.Trong lĩnh vực nghiên cứu nhận dạng tín hiệu, nhận dạng giọng nói. Trong sinh học, chuỗiMarkov được dùng để mã hoá, nghiên cứu về mối quan hệ giữa các vùng gen và dự đoán gen.Trong kĩ thuật mô phỏng, phương pháp Monte-Carlo; chuỗi Markov được đánh giá là một trongnhững phương pháp mô phỏng rất hữu hiệu bằng cách tạo ra những chuỗi số từ những số ngẫunhiên để phản ánh một cách chính xác các phân bố xác suất phức tạp, góp phần làm tăng tínhkhả thi của phương pháp Bayes. Đặc biệt trong lĩnh vực kinh tế, có khá nhiều ứng dụng dựatrên chuỗi Markov. Đề tài này trình bày các khái niệm và tính chất của chuỗi Markov và đề cập đến một sốứng dụng của chúng trong hoạt động kinh doanh, kinh tế thương mại như dự đoán các xuhướng phát triển của thị trường. Từ khóa - thời gian liên tục, không gian đếm được, thời gian rời rạc, thị trường, quá trìnhngẫu nhiên, ma trận chuyển tiếp. Abstract - Markov chains are an important mathematical tool in stochasticprocesses. The underlying idea is the Markov Property, in other words, that somepredictions about stochastic processes can be simplified by viewing the future asindependent of the past, given the present state of the process. This is used to simplifypredictions about the future state of a stochastic process. This research will begin with a brief introduction, followed by the analysis, and end withtips for further reading. The analysis will introduce the concepts of Markov chains, explaindifferent types of Markov Chains and present examples of its applications in finance. Keyword— continuous time, countable space, discrete time, market, stochastic process,transition matrix. MARKOV CHAINS AND THEIR APPLICATIONS TO PREDICT MARKET TREND I. INTRODUCTION 1) Definition 63 A Markov process is a stochastic process that satisfies the Markovproperty Markov. In simpler terms, a Markov process is a process for which one canmake predictions for its future based solely on its present state just as well as one couldknowing the processs full history. In other words, conditional on the present state of thesystem, its future and past states are independent. A Markov chain is a type of Markov process that has either a discrete state spaceor a discrete index set (often representing time), but the precise definition of a Markovchain varies. For example, it is common to define a Markov chain as a Markov processin either discrete or continuous time discrete or continuous time with a countable statespace (thus regardless of the nature of time), but it is also common to define a Markovchain as having discrete time in either countable or continuous state space. 2) Types of Markov chains The systems state space and time parameter index need to be specified. Thefollowing table gives an overview of the different instances of Markov processes fordifferent levels of state space generality and for discrete time and continuous time. Countable state space Continuous or general state spaceDiscrete time Markov chain on a Markov chain on a measure countable or finite state state space (for example, space Harris chain)Continuous time Continuous time Markov Any continuous stochastic process or Markov jump process with the Markov process property 3) Transitions The changes of state of the system are called transitions. The probabilitiesassociated with various state changes are called transition probabilities. The process ischaracterized by a state space, a transition matrix describing the probabilities ofparticular transitions, and an initial state (or initial distribution) across the state space.By convention, we assume all possible states and transitions have been included in thedefinition of the process, so there is always a next state, and the process does notterminate. A discrete-time random process invol ...
Nội dung trích xuất từ tài liệu:
Ứng dụng chuỗi Markov để dự đoán xu hướng của thị trường ỨNG DỤNG CHUỖI MARKOV ĐỂ DỰ ĐOÁN XU HƯỚNG CỦA THỊ TRƯỜNG Nguyễn Thị Hương Trường Đại học Hà Nội Tóm tắt - Khái niệm chuỗi Markov lần đầu tiên được đề cập bởi nhà Toán học người NgaA.Markov (1856 - 1922) vào năm 1906. Ngày nay chuỗi Markov được ứng dụng rộng rãi trongnhiều lĩnh vực khoa học khác nhau, như Thống kê, lý thuyết hàng chờ, logistics,…Chuỗi Markovthường được dùng để mô phỏng hệ thống phục vụ trong mối liên hệ với các tiến trình Poison.Trong lĩnh vực nghiên cứu nhận dạng tín hiệu, nhận dạng giọng nói. Trong sinh học, chuỗiMarkov được dùng để mã hoá, nghiên cứu về mối quan hệ giữa các vùng gen và dự đoán gen.Trong kĩ thuật mô phỏng, phương pháp Monte-Carlo; chuỗi Markov được đánh giá là một trongnhững phương pháp mô phỏng rất hữu hiệu bằng cách tạo ra những chuỗi số từ những số ngẫunhiên để phản ánh một cách chính xác các phân bố xác suất phức tạp, góp phần làm tăng tínhkhả thi của phương pháp Bayes. Đặc biệt trong lĩnh vực kinh tế, có khá nhiều ứng dụng dựatrên chuỗi Markov. Đề tài này trình bày các khái niệm và tính chất của chuỗi Markov và đề cập đến một sốứng dụng của chúng trong hoạt động kinh doanh, kinh tế thương mại như dự đoán các xuhướng phát triển của thị trường. Từ khóa - thời gian liên tục, không gian đếm được, thời gian rời rạc, thị trường, quá trìnhngẫu nhiên, ma trận chuyển tiếp. Abstract - Markov chains are an important mathematical tool in stochasticprocesses. The underlying idea is the Markov Property, in other words, that somepredictions about stochastic processes can be simplified by viewing the future asindependent of the past, given the present state of the process. This is used to simplifypredictions about the future state of a stochastic process. This research will begin with a brief introduction, followed by the analysis, and end withtips for further reading. The analysis will introduce the concepts of Markov chains, explaindifferent types of Markov Chains and present examples of its applications in finance. Keyword— continuous time, countable space, discrete time, market, stochastic process,transition matrix. MARKOV CHAINS AND THEIR APPLICATIONS TO PREDICT MARKET TREND I. INTRODUCTION 1) Definition 63 A Markov process is a stochastic process that satisfies the Markovproperty Markov. In simpler terms, a Markov process is a process for which one canmake predictions for its future based solely on its present state just as well as one couldknowing the processs full history. In other words, conditional on the present state of thesystem, its future and past states are independent. A Markov chain is a type of Markov process that has either a discrete state spaceor a discrete index set (often representing time), but the precise definition of a Markovchain varies. For example, it is common to define a Markov chain as a Markov processin either discrete or continuous time discrete or continuous time with a countable statespace (thus regardless of the nature of time), but it is also common to define a Markovchain as having discrete time in either countable or continuous state space. 2) Types of Markov chains The systems state space and time parameter index need to be specified. Thefollowing table gives an overview of the different instances of Markov processes fordifferent levels of state space generality and for discrete time and continuous time. Countable state space Continuous or general state spaceDiscrete time Markov chain on a Markov chain on a measure countable or finite state state space (for example, space Harris chain)Continuous time Continuous time Markov Any continuous stochastic process or Markov jump process with the Markov process property 3) Transitions The changes of state of the system are called transitions. The probabilitiesassociated with various state changes are called transition probabilities. The process ischaracterized by a state space, a transition matrix describing the probabilities ofparticular transitions, and an initial state (or initial distribution) across the state space.By convention, we assume all possible states and transitions have been included in thedefinition of the process, so there is always a next state, and the process does notterminate. A discrete-time random process invol ...
Tìm kiếm theo từ khóa liên quan:
Thời gian liên tục Không gian đếm được Thời gian rời rạc Ma trận chuyển tiếp Lý thuyết hàng chờ Phương pháp BayesTài liệu liên quan:
-
7 trang 50 0 0
-
Ebook Phân tích dữ liệu với R: Phần 2
262 trang 32 0 0 -
Ảnh hưởng của giá dầu đến Z-score của các ngân hàng thương mại tại Việt Nam: Phương pháp Bayes
12 trang 29 0 0 -
PHÂN LOẠI BẰNG PHƯƠNG PHÁP BAYES TỪ SỐ LIỆU RỜI RẠC
10 trang 18 0 0 -
8 trang 16 0 0
-
Hướng dẫn ứng dụng phân tích dữ liệu với R: Phần 2
285 trang 15 0 0 -
6 trang 13 0 0
-
Vai trò của văn hóa đối với phát triển kinh tế: Bằng chứng từ một số nước Châu Á
14 trang 12 0 0 -
Tác động của đầu tư trực tiếp nước ngoài đến phát triển bền vững tại Việt Nam
5 trang 10 0 0 -
Đánh giá khả năng trả nợ vay của khách hàng bằng các phương pháp phân loại
8 trang 10 0 0