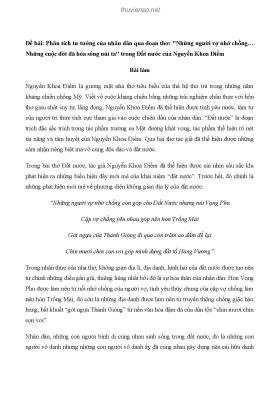
Xây dựng mô hình tối ưu để dự báo chiến lược maketing tại ngân hàng
Số trang: 6
Loại file: pdf
Dung lượng: 168.77 KB
Lượt xem: 16
Lượt tải: 0
Xem trước 2 trang đầu tiên của tài liệu này:
Thông tin tài liệu:
Mục tiêu của bài viết này là đề xuất một mô hình bỏ phiếu để phân loại dữ liệu maketing ngân hàng. Mô hình bỏ phiếu được xây dựng từ ba mô hình phân loại trí tuệ nhân tạo nổi tiếng, bao gồm máy học vectơ hỗ trợ (SVM), Navie Bayes (NB) và Cây quyết định (DT). Mời các bạn cùng tham khảo!
Nội dung trích xuất từ tài liệu:
Xây dựng mô hình tối ưu để dự báo chiến lược maketing tại ngân hàng INTERNATIONAL CONFERENCE FOR YOUNG RESEARCHERS IN ECONOMICS & BUSINESS 2020 ICYREB 2020 DEVELOPING VOTING - ENSEMBLE MODEL FOR BANK MAKETING PREDICTION XÂY DỰNG MÔ HÌNH TỐI ƯU ĐỂ DỰ BÁO CHIẾN LƯỢC MAKETING TẠI NGÂN HÀNG Pham Thi Phuong Trang, MA University of Technology and Education - The University of Danang trangpham3112@gmail.com Abstract Maketing provides a link between the banks’ activities to the market and contributes to cre- ating a competitive position of the bank. Therefore, creating an intelligent model that can accu- rately predict bank maketing campaign has always been a great interest for investors and financial analysts. The objective of this paper is to propose a model-voting to classify bank maket- ing dataset. The voting model is constructed from three well-known individual artificial intelligent classification models, including Support vector machine (SVM), Navie Bayes (NB) and Decision Tree (DT). Analytical results showed the voting model was superior to other comparative models for bank maketing dataset. Particularly, the SVM - NB - DT was the best voting model achieving the highest result with 89.957% of accuracy. Moreover, other voting models as SVM-NB, NB-DT aslo had good results with 88.975% and 88.931% of accuracy, respectively. Therefore, voting- ensemble model is considered a suitable tool to predict bank maketing dataset. Keywords: Bank maketing, Decision Tree, Navie Bayes, Support vector machine, voting model. Tóm tắt Maketing cung cấp mối liên kết giữa các hoạt động của ngân hàng với thị trường và góp phần tạo ra vị thế cạnh tranh cho ngân hàng đó. Vì vậy, việc tạo ra một mô hình thông minh có thể dự báo một cách chính xác chiến lược maketing ngân hàng luôn là mối quan tâm lớn đối với các nhà đầu tư và các nhà phân tích tài chính. Mục tiêu của bài viết này là đề xuất một mô hình bỏ phiếu để phân loại dữ liệu maketing ngân hàng. Mô hình bỏ phiếu được xây dựng từ ba mô hình phân loại trí tuệ nhân tạo nổi tiếng, bao gồm máy học vectơ hỗ trợ (SVM), Navie Bayes (NB) và Cây quyết định (DT). Kết quả phân tích cho thấy mô hình bỏ phiếu là vượt trội so với các mô hình so sánh khác với bộ dữ liệu maketing ngân hàng. Đặc biệt, SVM - NB - DT là mô hình bỏ phiếu tốt nhất đạt kết quả cao nhất với 89.957% độ chính xác. Ngoài ra, các mô hình bỏ phiếu khác như SVM-NB, NB-DT cũng đạt kết quả khá tốt với độ chính xác lần lượt là 88.975% và 88.931%. Do đó, mô hình tập hợp bỏ phiếu được coi là một công cụ phù hợp để dự đoán bộ dữ liệu maketing ngân hàng. Từ khóa: Maketing ngân hàng, Cây quyết định, Navie Bayes, Máy học véc tơ hỗ trợ, mô hình bỏ phiếu. 592 INTERNATIONAL CONFERENCE FOR YOUNG RESEARCHERS IN ECONOMICS & BUSINESS 2020 ICYREB 2020 1. Introduction Bank maketing plays a vital role in establishing beneficial relationships among stakehold- ers. Bank maketing can be considered as the bank’s efforts to satisfy customer needs and realize profit goals. Therefore, the bank needs to orient the operations of its departments and the entire banking staff to create, maintain and develop relationships with customers - the factors that de- termine its survival. Thus, a wide range of methods have been proposed to improve the bank maketing cam- paigns. For example, Hu used data mining (DT) in analyzing retailing bank customer attrition (Hu 2005) or Li et al. also applied DT in predicting Credit Card Customer Segmentation and Tar- get Marketing Based (Li, Wu et al. 2011). Moreover, several works used a classification DM ap- proach to build a predictive model that can label a data item into binary classes (Abbas 2015, Asif 2018). Several DM algorithms can be used for classifying marketing contacts, each one with its own purposes and capabilities. Examples of popular DM techniques are: Naïve Bayes (NB), Decision Trees (DT) and Support Vector Machines (SVM). NB, DT and SVM are individual well-know models in classifying and were apllied in many works. For example, the SVM-based classification model has been used to forecast soil quality (Liu, Wang et al. 2016), relevance vector regression (RVR) and the SVM have been used to predict the Rock Mass Rating of tunnel host rocks (Gholami, Rasouli et al. 2013). Jiangtao Ren et.al proposed a novel Naive Bayes clas- sification algorithm for uncertain data (Ren, Lee et al. 2009). Moreover, many researchers used Naive Bayes model to classify Web Documents (Ren, Lee et al. 2009), Text document (Zhang and Gao 2011). With the popular application of SVM, NB and DT in classification problm ...
Nội dung trích xuất từ tài liệu:
Xây dựng mô hình tối ưu để dự báo chiến lược maketing tại ngân hàng INTERNATIONAL CONFERENCE FOR YOUNG RESEARCHERS IN ECONOMICS & BUSINESS 2020 ICYREB 2020 DEVELOPING VOTING - ENSEMBLE MODEL FOR BANK MAKETING PREDICTION XÂY DỰNG MÔ HÌNH TỐI ƯU ĐỂ DỰ BÁO CHIẾN LƯỢC MAKETING TẠI NGÂN HÀNG Pham Thi Phuong Trang, MA University of Technology and Education - The University of Danang trangpham3112@gmail.com Abstract Maketing provides a link between the banks’ activities to the market and contributes to cre- ating a competitive position of the bank. Therefore, creating an intelligent model that can accu- rately predict bank maketing campaign has always been a great interest for investors and financial analysts. The objective of this paper is to propose a model-voting to classify bank maket- ing dataset. The voting model is constructed from three well-known individual artificial intelligent classification models, including Support vector machine (SVM), Navie Bayes (NB) and Decision Tree (DT). Analytical results showed the voting model was superior to other comparative models for bank maketing dataset. Particularly, the SVM - NB - DT was the best voting model achieving the highest result with 89.957% of accuracy. Moreover, other voting models as SVM-NB, NB-DT aslo had good results with 88.975% and 88.931% of accuracy, respectively. Therefore, voting- ensemble model is considered a suitable tool to predict bank maketing dataset. Keywords: Bank maketing, Decision Tree, Navie Bayes, Support vector machine, voting model. Tóm tắt Maketing cung cấp mối liên kết giữa các hoạt động của ngân hàng với thị trường và góp phần tạo ra vị thế cạnh tranh cho ngân hàng đó. Vì vậy, việc tạo ra một mô hình thông minh có thể dự báo một cách chính xác chiến lược maketing ngân hàng luôn là mối quan tâm lớn đối với các nhà đầu tư và các nhà phân tích tài chính. Mục tiêu của bài viết này là đề xuất một mô hình bỏ phiếu để phân loại dữ liệu maketing ngân hàng. Mô hình bỏ phiếu được xây dựng từ ba mô hình phân loại trí tuệ nhân tạo nổi tiếng, bao gồm máy học vectơ hỗ trợ (SVM), Navie Bayes (NB) và Cây quyết định (DT). Kết quả phân tích cho thấy mô hình bỏ phiếu là vượt trội so với các mô hình so sánh khác với bộ dữ liệu maketing ngân hàng. Đặc biệt, SVM - NB - DT là mô hình bỏ phiếu tốt nhất đạt kết quả cao nhất với 89.957% độ chính xác. Ngoài ra, các mô hình bỏ phiếu khác như SVM-NB, NB-DT cũng đạt kết quả khá tốt với độ chính xác lần lượt là 88.975% và 88.931%. Do đó, mô hình tập hợp bỏ phiếu được coi là một công cụ phù hợp để dự đoán bộ dữ liệu maketing ngân hàng. Từ khóa: Maketing ngân hàng, Cây quyết định, Navie Bayes, Máy học véc tơ hỗ trợ, mô hình bỏ phiếu. 592 INTERNATIONAL CONFERENCE FOR YOUNG RESEARCHERS IN ECONOMICS & BUSINESS 2020 ICYREB 2020 1. Introduction Bank maketing plays a vital role in establishing beneficial relationships among stakehold- ers. Bank maketing can be considered as the bank’s efforts to satisfy customer needs and realize profit goals. Therefore, the bank needs to orient the operations of its departments and the entire banking staff to create, maintain and develop relationships with customers - the factors that de- termine its survival. Thus, a wide range of methods have been proposed to improve the bank maketing cam- paigns. For example, Hu used data mining (DT) in analyzing retailing bank customer attrition (Hu 2005) or Li et al. also applied DT in predicting Credit Card Customer Segmentation and Tar- get Marketing Based (Li, Wu et al. 2011). Moreover, several works used a classification DM ap- proach to build a predictive model that can label a data item into binary classes (Abbas 2015, Asif 2018). Several DM algorithms can be used for classifying marketing contacts, each one with its own purposes and capabilities. Examples of popular DM techniques are: Naïve Bayes (NB), Decision Trees (DT) and Support Vector Machines (SVM). NB, DT and SVM are individual well-know models in classifying and were apllied in many works. For example, the SVM-based classification model has been used to forecast soil quality (Liu, Wang et al. 2016), relevance vector regression (RVR) and the SVM have been used to predict the Rock Mass Rating of tunnel host rocks (Gholami, Rasouli et al. 2013). Jiangtao Ren et.al proposed a novel Naive Bayes clas- sification algorithm for uncertain data (Ren, Lee et al. 2009). Moreover, many researchers used Naive Bayes model to classify Web Documents (Ren, Lee et al. 2009), Text document (Zhang and Gao 2011). With the popular application of SVM, NB and DT in classification problm ...
Tìm kiếm theo từ khóa liên quan:
Tạp chí Khoa học Chiến lược maketing ngân hàng Phân loại dữ liệu maketing Mô hình bỏ phiếu Cây quyết địnhTài liệu liên quan:
-
6 trang 307 0 0
-
Thống kê tiền tệ theo tiêu chuẩn quốc tế và thực trạng thống kê tiền tệ tại Việt Nam
7 trang 273 0 0 -
5 trang 234 0 0
-
10 trang 222 0 0
-
8 trang 220 0 0
-
Khảo sát, đánh giá một số thuật toán xử lý tương tranh cập nhật dữ liệu trong các hệ phân tán
7 trang 217 0 0 -
Quản lý tài sản cố định trong doanh nghiệp
7 trang 208 0 0 -
Khách hàng và những vấn đề đặt ra trong câu chuyện số hóa doanh nghiệp
12 trang 208 0 0 -
6 trang 207 0 0
-
Nâng cao hiệu quả tra cứu ảnh nhãn hiệu sử dụng cây quyết định và phản hồi liên quan
10 trang 174 0 0